Our team is here to help you choose the right program for your life and your goals.
- How to Apply
- Transfer Credits
- Prospective Students & Parents
- Military Admissions

Master’s title
- Master of Science in Cybersecurity
Bachelor's title
- Accelerated Cyber and Information Security Technology
- Cloud Computing
- Cyber and Information Security Technology
- Cybersecurity
- Data Analytics
- Digital Forensics Technology
- Mobile Development
- Software Development
- Web Design and Development
- Systems Engineering Master's - Mechatronics
- Electronic Systems Engineering Technology
- Electronics Engineering Technology
- Mechanical Engineering Technology
- Mechatronics (ESET)
- Mechatronics
Associate’s title
- Computer-Aided Drafting and Design
- Family Nurse Practitioner (MSN)
- Nursing Education (MSN)
- Bachelor to BSN Nursing Program (BS to BSN)
- Bachelor of Science in Nursing (RN to BSN)
- Associates Degree in Nursing (ADN)
Diploma title
- Practical Nursing (PN)
- Master's in Healthcare Administration
- Healthcare Administration
- Dental Assistant
- Medical Assisting
- Paramedic (EMT)
- Radiologic Sciences
- Diagnostic Medical Sonography
- Physical Therapist Assistant
- Radiography
- Surgical Technology
- Master of Business Administration (MBA)
- Business Analytics
- Business Management
- General Management
- Hospitality Management
- IT Management
- Operations, Logistics, and Supply Chain Management
- Management Master's - Human Resources Management
- Management Master's - Organizational Leadership
- Human Resources Management
- Project Management
- Crime and Intelligence Analysis
- Criminal Justice
- Digital Forensics
- Homeland Security
- Food Service Management
- Baking and Pastry Arts
- Culinary Arts
- Culinary Nutrition
- Electronic Engineering Technology
- RN to BSN Program
- Master of Business Administration
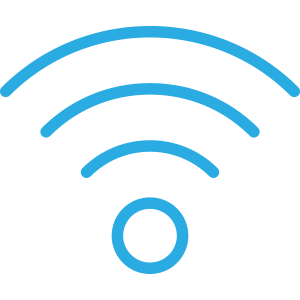
- Virginia Beach
- Newport News
- Northern Virginia
- Orlando Lake Mary
- San Antonio
About ECPI University
Founded in 1966, ECPI University promotes a student-centered learning environment as well as personal and professional development through education.
- Why Choose ECPI
- Mission & Values
- Message from the President
- Accreditation
- Academic Calendar
- Course Catalog
- Career Services
- Bachelor's
- Associate’s
Find the right degree program for you.
- Health Science
- By Degree Level
- Master's
- Associate's & Diplomas
- By Location
- Information Technology
- Engineering Technology
- Explore All Technology Programs
- Bachelor of Science in Nursing (Accelerated BSN)
- Explore Our Nursing Programs
- Health Sciences
- Advanced Clinical
- Explore Our Health Science Programs
- General Business Management
- Master of Human Resources Management
- Master of Organizational Leadership
- Explore Our Business Programs
- Explore Our Criminal Justice Programs
- Explore Our Culinary Arts Programs
- North Carolina
- South Carolina
Tuition and Financial Assistance
At ECPI University, an affordable education is within reach. Financial assistance options are available to help you invest in your future.
- How to Pay for College
- Tuition & Fees
- Scholarships
- VA & Military Benefits
- Employer Partnership Program
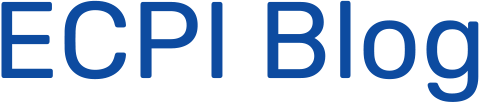
Statistics is a vital part of human knowledge but most student nurses dread quantitative research and statistics in particular. Nursing practice is mostly based on empirical evidence . Evidence-based-practice requires clinicians and nurses to read literature that consists of quantitative research reports.
Although nursing often includes prevention of illnesses, promotion of health and caring for the sick, it also involves statistical skills such as measurements, drawing, and interpreting charts and diagrams.

Human beings respond to exposure to various risk factors, diseases, and treatments differently. Therefore, all nurses should be able to summarize their findings, interpret results, and display health care data in the right ways.
How Nurses Use Statistics in their Job
Enhance patient care: Through statistics, nurses are able to prioritize treatments and determine whether a patient needs immediate medical attention or follow-up care. As a nurse, you will use statistics to identify specific patterns in important signs and symptoms and be able to respond better to any medical changes to your patient. Using frequency charts or data sheets to document the timing of medication given to patients is another way that nurses use statistics.
Support evidence-based nursing practices: The nursing profession is mostly based on empirical evidence that establishes effective protocols for patient care. However, for evidence-based practice to be well established in the nursing profession , nurses need basic statistics understanding to be able to read, comprehend, and interpret relevant literature.
Compare options for nursing practice: Nurses use statistics to determine whether the nursing interventions of their choice are effective. For instance, through statistics, nurses are able to tell that neither replacing intravenous fluids, nor administration sets can reduce infections. In some settings, nurses have to make several decisions during critical care and statistics knowledge comes in handy. Experience and emotional instinct aren’t enough to help make the right decisions.
The U.S. health care system is set to undergo profound changes to improve patient care. For this reason, formal nursing education is essential and its main goal is to prepare nurses to meet patients’ needs, advance science, and to deliver safe and quality patient care. Other important reasons for formal nursing education include:
Healthcare has become more complex and challenging: Nursing has become more challenging due to the plethora of electronic information and published literature that exists. Additionally, new knowledge meant to help improve patient care is always emerging, which means that a nurse is never finished learning.
Healthcare leadership demands knowledgeable and competent staff: Formal nursing education is an important component of the commitment to become a leader in the nursing profession. For one to become a nursing leader, one must be able to keep up with the current trends, be able to solve nursing related problems, and know about new technology that can improve patient care.
Formal education is a mandatory requirement to become a nurse: For you to become a registered nurse, you will need to successfully complete a formal nursing education program to qualify for the NCLEX-RN (National Council Licensure Examination for Registered Nurses) exam to acquire your nursing license. Keep in mind that for you to practice nursing, you must be a licensed nurse , and one of the requirements is passing a state-approved nursing training program.
Nursing School
Many who aspire to join this profession often ask “how hard is nursing school?” Nursing requires you to go to school and get licensed. Nursing school can be challenging because as a nurse, people will count on you to save lives.
However, nursing school isn’t all about studying. For most students, it’s a mixture of hard work, supportive camaraderie, and fun. Studying hard in nursing school helps you discover what you’re capable of achieving. This is also where you will start to grow into a well-respected and admirable professional.
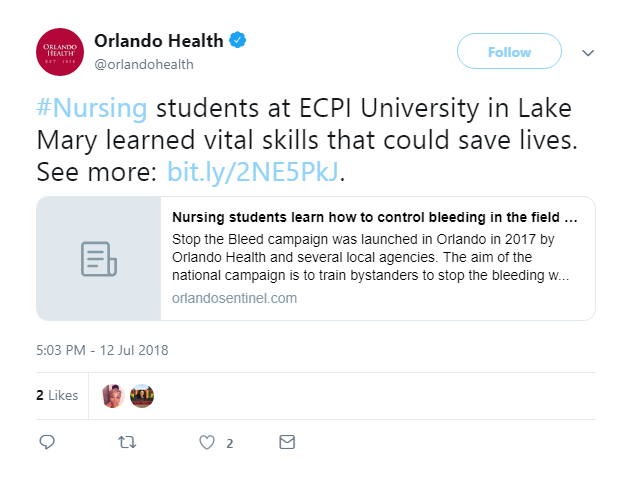
Are you interested in a future as a nurse? If you want to earn an Associate Degree of Nursing , ECPI University offers this degree at an accelerated pace. For more information on this exciting opportunity, connect with a friendly ECPI University admissions counselor today .
It could be the Best Decision You Ever Make !
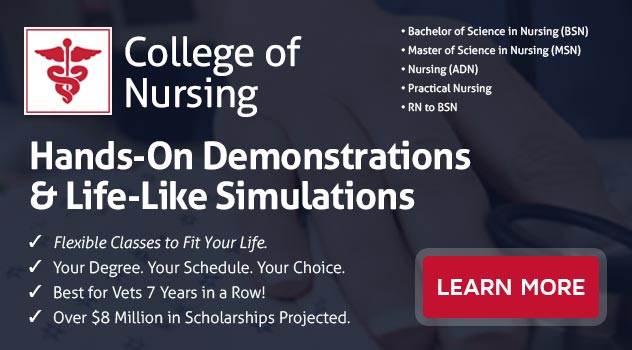
DISCLAIMER – ECPI University makes no claim, warranty, or guarantee as to actual employability or earning potential to current, past or future students or graduates of any educational program we offer. The ECPI University website is published for informational purposes only. Every effort is made to ensure the accuracy of information contained on the ECPI.edu domain; however, no warranty of accuracy is made. No contractual rights, either expressed or implied, are created by its content.
For more information about ECPI University or any of our programs click here: http://www.ecpi.edu/ or http://ow.ly/Ca1ya .
View the discussion thread.
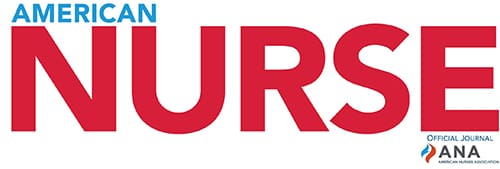
Research 101: Descriptive statistics
Use these tools to analyze data vital to practice-improvement projects..
By Brian Conner, PhD, RN, CNE and Emily Johnson, PhD
- Nurses at every level should be able to understand and apply basic statistical analyses related to performance improvement projects.
- Measures of central tendency (such as mean) and variability (such as standard deviation) are fairly common and easy to use.
How many times have you said (or heard), “Statistics are too complicated”? A significant percentage of graduate students and nurses in clinical practice report feeling anxious when working with statistics. And although some statistical analysis is pretty complicated, you don’t need a doctoral degree to understand and use descriptive statistics.
What are descriptive statistics?

Sometimes, descriptive statistics are the only analyses completed in a research or evidence-based practice study; however, they don’t typically help us reach conclusions about hypotheses. Instead, they’re used as preliminary data, which can provide the foundation for future research by defining initial problems or identifying essential analyses in more complex investigations.
Common descriptive statistics
The most common types of descriptive statistics are the measures of central tendency (mean, median, and mode) that are used in most levels of math, research, evidence-based practice, and quality improvement. These measures describe the central portion of frequency distribution for a data set.
The most familiar of these is the mean , or average, which most people use and understand. It’s calculated by adding the sum of values in the data and dividing by the total number of observations.
The median is a number found at the exact middle of a set of data. If there are two numbers at the middle of the data set (which occurs when there is an even number of data points), these two numbers are averaged to identify the median. It’s typically used to describe a data set that has extreme outliers (very low or very high numbers, distant from the majority of data points), in which case the mean will not accurately represent the data. (See What to do with outliers .) To calculate a mean or median, data must be quantitative/continuous (have an infinite number of possibilities).
What to do with outliers
When analyzing descriptive statistics, watch for outliers. These data points are distant from the majority of observations and may be the result of measurement error, coding error, or extreme variability in an observation. In addition to visually perusing data for outliers, you can identify them using graphical display and complex modeling. Depending on the number of outliers, they’re either statistically transformed (using a complex statistical formula to balance all variable values) or excluded from the data set. |
The mode represents the most frequently occurring number or item in a data set. Some data sets have more than one mode, making them bimodal (two modes) or multimodal (more than two modes). The mode can be calculated with data that are quantitative/continuous or qualitative/categorical (have a finite number of categories or groups, such as sex, race, or education level). The mode is the only measure of central tendency that can be analyzed with qualitative/categorical data.
Less common descriptive statistics
Measures of variability or dispersion are less common descriptive statistics, but they’re still important because they describe the spread of values across a data set. Although the central tendency of data is vital, the range of values (the difference between the maximum and minimum values in the data) also may be important to note. The range not only sets boundaries for your data set and indicates the spread, but it also can identify errors in the data. For example, if you have a data set with a diastolic blood pressure range of 230 (highest diastolic value) to 25 (lowest diastolic value) = 205 (range), an error probably exists in your data because the values of 230 and 25 aren’t valid blood pressure measures in most studies. Other measures of variability include standard deviation, variance, and quartiles. (See Other variability measures .)
Other variability measures
Standard deviation, variance, and quartile can be used in addition to range to measure variability of data. is the average distance of each data point from the mean of the data set. It’s calculated by taking the square root of the sum of all numbers minus the mean (squared) and dividing by one less than the number of values. For example, in a data set of five systolic blood pressures of 125, 128, 142, 145, and 150, the mean would be 138, based on this calculation: (125+128+142+145+150)/5. The standard deviation would be 10.9, based on this calculation: √(((125-138)2 + (128-138)2 + (142-138)2 + (145-138)2 + (150-138)2)/(5-1)), indicating that there’s not a large dispersion in this set of systolic measures. (Don’t worry about the complexity of the formula; you can enter the data points in a that does the calculation for you. The formula is here to illustrate the point.) The also describes the variation of data points from the mean, but it’s affected by outliers. If the standard deviation and variance are large, the spread of data points in the data set also is large; however, if the standard deviation and variance are small, most data points are close to the mean. Whether standard deviation and variance are determined to be small or large depends on the range of data. For example, in data with a range of 5, a standard deviation of 4 would be large; however, in data with a range of 10,000, a standard deviation of 4 would be small. A (q) consists of three points, q1 (lower), q2 (median), and q3 (upper), that divide a list of numbers into four equal categories. When using quartiles, you can identify the interquartile range (q3-q1), which describes the middle part of the data set. Range, standard deviation, variance, and quartiles are all used with quantitative/continuous data, but they can’t be used to analyze qualitative/categorical data. |
Practical application of descriptive statistics
To put all of this information into perspective, here’s an example of how these measures can be used in a clinical setting.
A rural primary care clinic has a high percentage of patients with diabetes whose glycated hemoglobin (HbA1c) levels are greater than 7% (uncontrolled HbA1c) and body mass index (BMI) is over 30. The clinic implements a 9-month quality-improvement initiative to lower these numbers. The initiative includes a wellness education program focused on exercise, healthy eating, and understanding the importance of regular blood glucose monitoring. Before implementing the program, the clinic collects 3 months of aggregate data (3, 6, and 9 months before the intervention) for all patients with diabetes in the clinic, including HbA1c levels, BMIs, and patients with uncontrolled HbA1c. Gender and age also are collected. The clinic then collects the same data 3, 6, and 9 months after implementation of the program. (See Snapshot of aggregate data .) Because of the different types of data collected, different measures of central tendency and variability can help describe outcomes. (See Statistical analysis examples .)
Snapshot of aggregate data

Statistical analysis examples

Implications for practice
Nurses are increasingly asked to participate and lead evidence-based practice and quality-improvement projects. Many healthcare organizations, including those aspiring to or holding Magnet® recognition from the American Nurses Credentialing Center, require that nurses take part in these activities to achieve higher levels of professional development within clinical ladder programs. Nurses can and should learn how to use descriptive statistics to analyze and depict vital data related to practice-improvement projects.
Brian Conner is adjunct faculty at the School of Nursing and Health Sciences for Simmons College in Boston, Massachusetts. Emily Johnson is an assistant professor at the Medical University of South Carolina College of Nursing in Charleston.
Selected references
American Nurses Credentialing Center (ANCC). Magnet Recognition Program® Overview . 2016.
Heavey E. Statistics for Nursing: A Practical Approach . 2nd ed. Burlington, MA: Jones and Bartlett Learning; 2015.
Thabane L, Akhtar-Danesh N. Guidelines for reporting descriptive statistics in health research. Nurse Res . 2008;15(2):72-81.
Zhang Y, Shang L, Wang R, et al. Attitudes toward statistics in medical postgraduates: Measuring, evaluating and monitoring. BMC Med Educ . 2012;12:117.
1 Comment .
This is a great data set and I would like to see if I can have copy right to use it in my « statistical thinking for Nursing » course here at Castleton Unvesity to demonstrate Bootsrapjng technique as Inferential statistics. Please let me know. Thank You Dr. Rajia
Comments are closed.
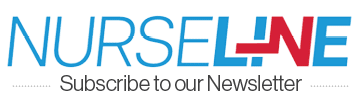
NurseLine Newsletter
- First Name *
- Last Name *
- Hidden Referrer
*By submitting your e-mail, you are opting in to receiving information from Healthcom Media and Affiliates. The details, including your email address/mobile number, may be used to keep you informed about future products and services.
Test Your Knowledge
Recent posts.

Interpreting statistical significance in nursing research

Introduction to qualitative nursing research
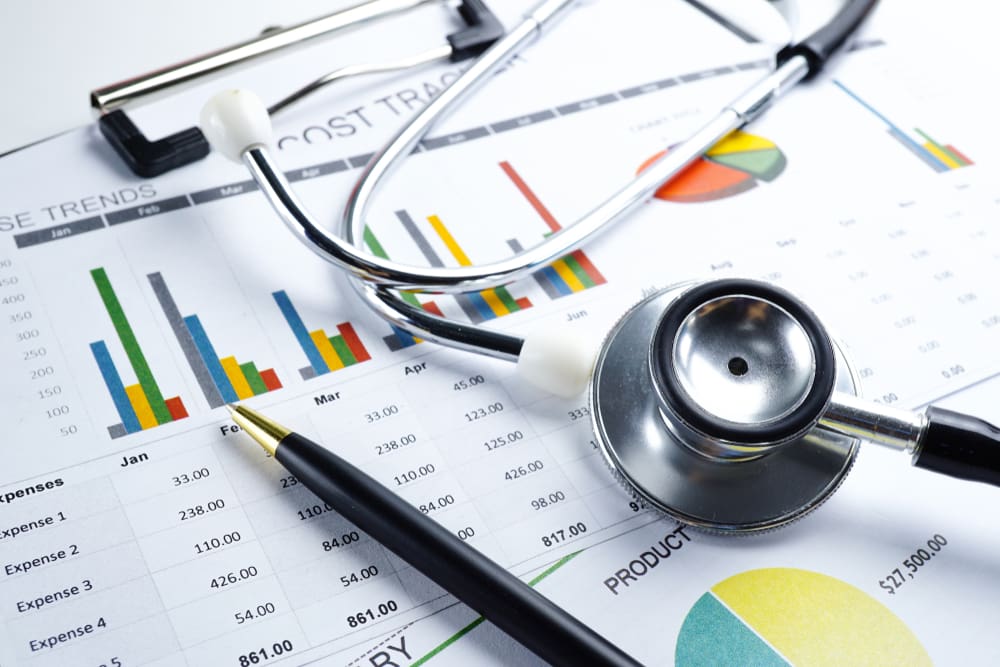
Navigating statistics for successful project implementation
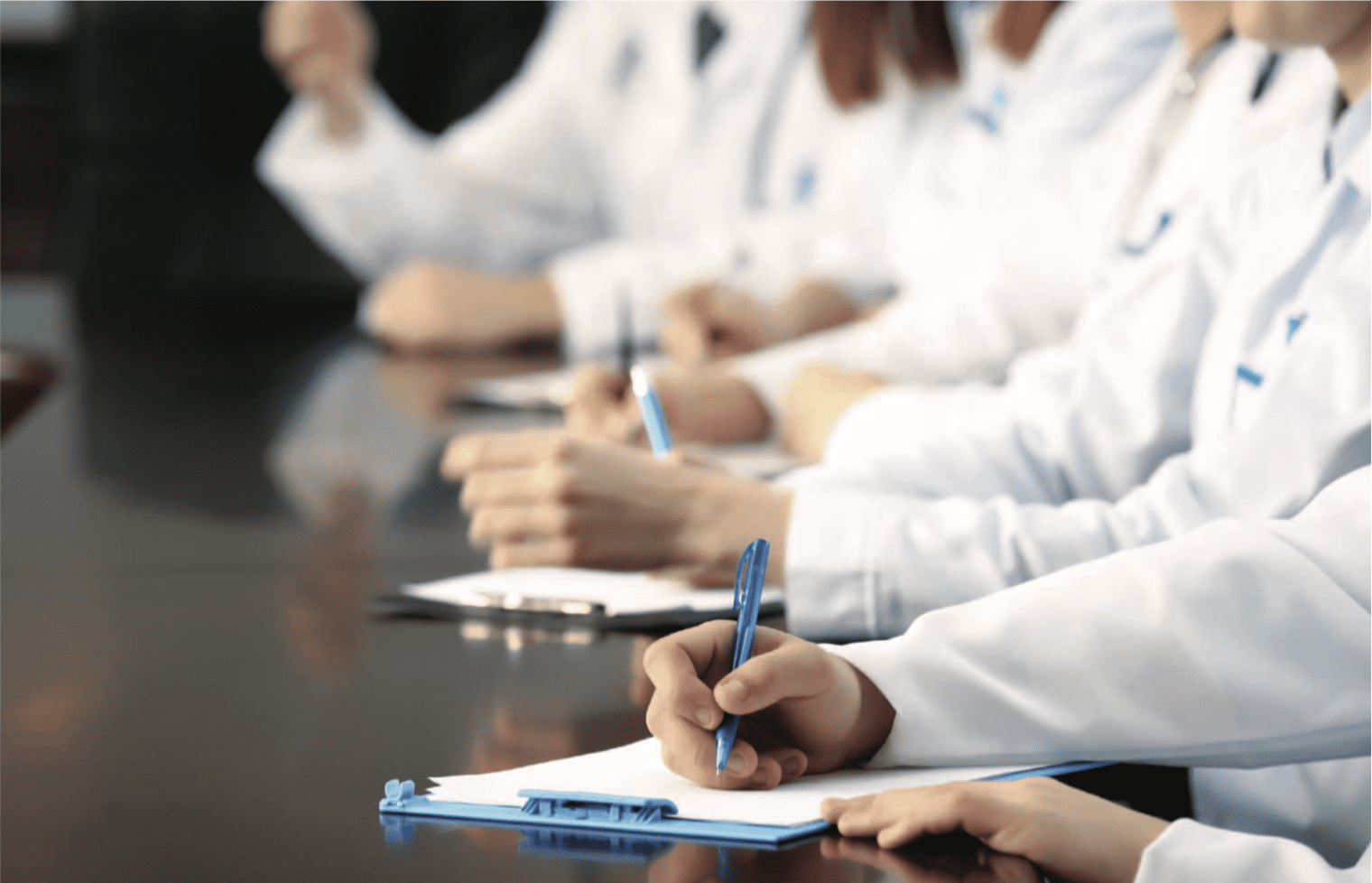
Nurse research and the institutional review board
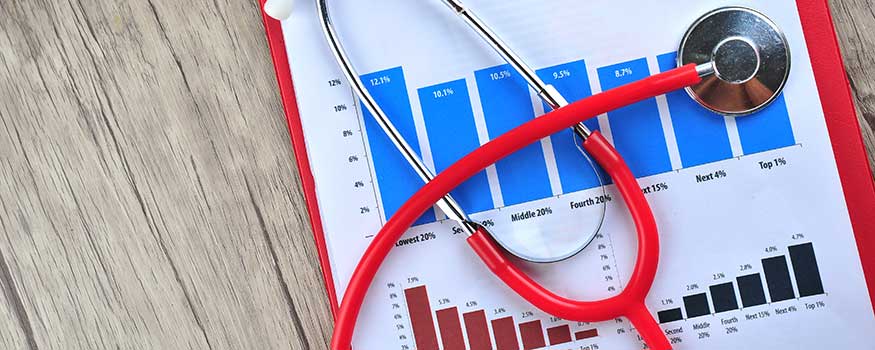
Research 101: Forest plots
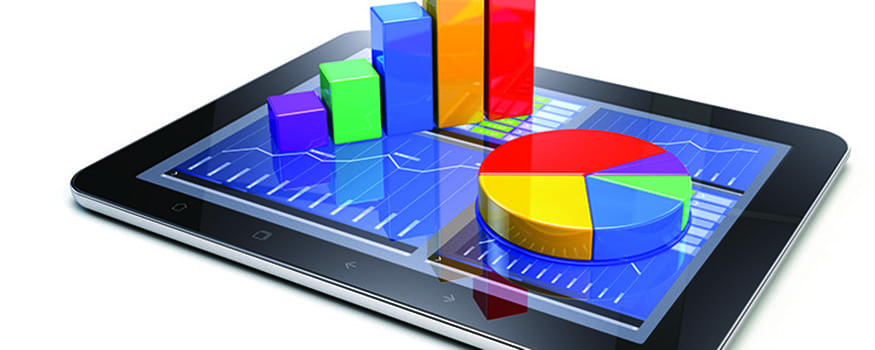
Understanding confidence intervals helps you make better clinical decisions
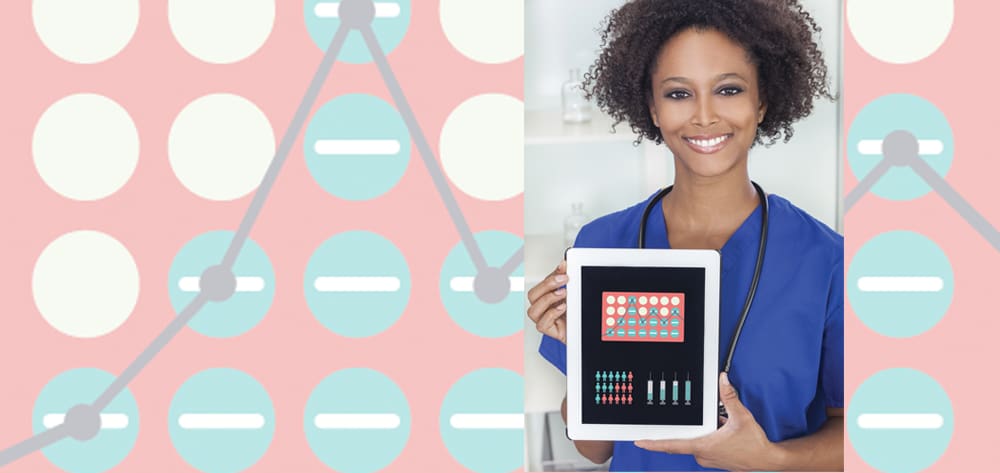
Differentiating statistical significance and clinical significance

Differentiating research, evidence-based practice, and quality improvement
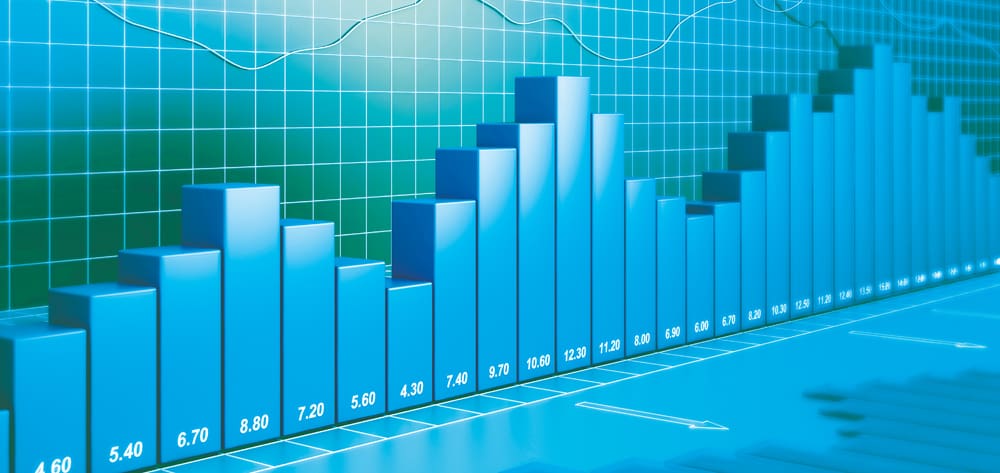
Are you confident about confidence intervals?
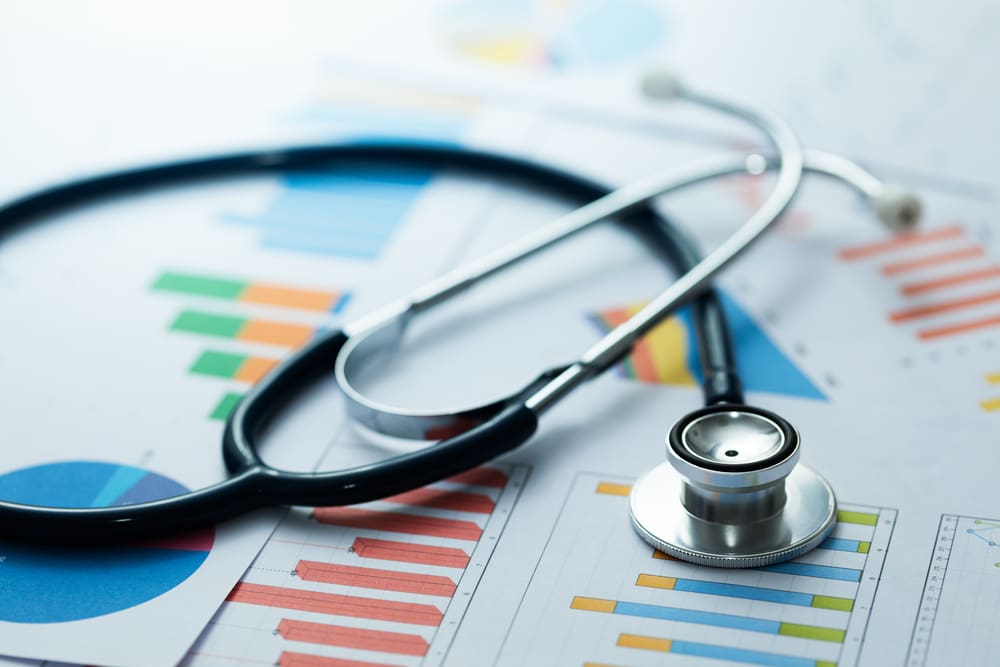
Making sense of statistical power
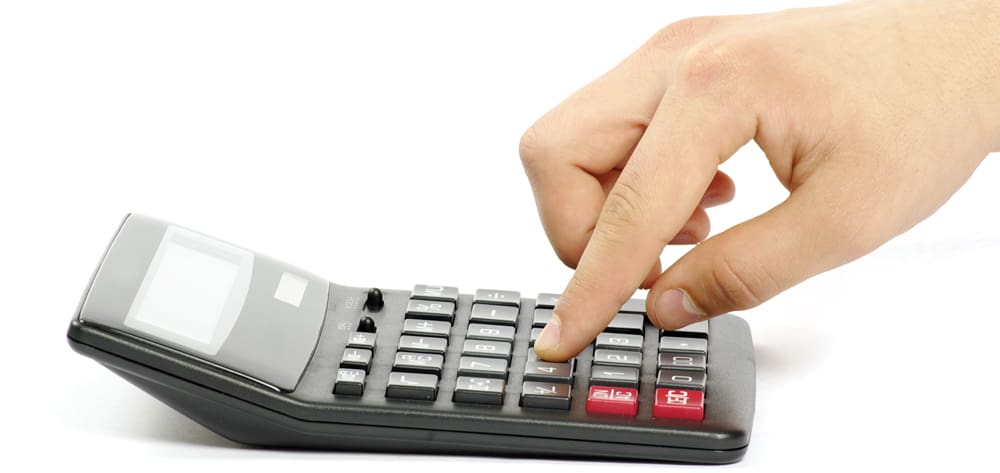
The P value: What it really means
- Call to +1 844 889-9952
Application of Statistics in Health Care – Importance & Examples of Use
📄 Words: | 875 |
---|---|
📝 Subject: | |
📑 Pages: | 4 |
✍️ Type: | Essay |
Wondering how are statistics used in healthcare? On this page, you will find out how the public health sector collects and analyzes statistical data, including using machine learning algorithms. One of the applications of such a method is processing large amounts of data and making predictions. Additionally, the text highlights some challenges and opportunities in using statistical data in healthcare.
- The Importance of Statistics in Healthcare
- Machine Learning in Diagnosis
- Statistics in Healthcare Examples
Video Voice-over
Exploring the importance of statistics in healthcare.
Data and its’ interpretation are vital for statistical application in the field of health care. Nowadays, healthcare systems carry a significant amount of data on patients, including their demographics, treatment plans, methods of payment, and insurance information. This essay will describe the standard application of statistics in health care, and discuss its significance and utilization of statistical knowledge in nurses’ work in a hospital.
The healthcare system’s information on the patients, treatment programs, and payment process could be systematized and organized in the form of statistics. Statistics health care data could be applied in a number of various cases, like providing a view of cancer statistics and how they relate to specific demographics or machine learning algorithms that predict diabetes diagnosis (Islam et al., 2018). The commonly used statistical models are now also used in the machine learning process.
The Use of Machine Learning in Diagnosis
Machine learning helps with the fast analysis of a massive amount of data that humans cannot process. For example, the popular algorithm used by Netflix and Amazon that compares the customers and recommends movies and books for customers that expressed similar interests was used to predict patients’ responses or risk of disease (Islam et al., 2018). The method introduces a new approach to the patients’ personal care, and it is not the only way in which machine learning could be helpful. Machine learning is used as an alternative to costly diagnostic methods for Coronary Artery Disease (CAD), and the results’ level of accuracy is almost equal (Islam et al., 2018). The application of machine learning to Emergency Care is used in event stimulations to improve the Emergency Department operations and provide effective communication and flow in staff groups.
The ability for machine learning appeared as a consequence of a large amount of collected clinical data from the last years. The combination of large amounts of health care data with faster computers further resulted in the form of deep-learning models, which could, for example, detect diabetic retinopathy from retina photos without human interventions (Beam et al., 2018). The process of data collecting includes not only the health care records but also a variety of sources like fitness trackers, genetic testing, etc. Considering nurses’ work in a hospital, statistical knowledge is essential and is utilized frequently in the work process. The data is obtained through interviews with patients; moreover, apart from the facts like age and blood type, nurses ask the patients to provide information about their health habits and close relatives’ health history. The collected data is used to ensure a correct diagnosis and impacts the process of decision-making in terms of treatment methods and the application of specific frameworks. The statistical knowledge used by nurses in day-to-day operations implies knowledge of health risks for certain age groups and making decisions in treatments based on their effectiveness.
How Are Statistics Used in Healthcare
The evaluation of large amounts of data health care workers encounter, like patients’ previous use of medications, and earlier admissions to hospitals is eased by using machines to store the data and help with decision-making. The application of statistical data to health care provides quality health care services by ensuring that none of the valid information is missing. In the same way, the application of statistical data offers safety for the patients. The research shows that statistical data could be used to measure drug safety alert response and defect adverse drug reactions to ensure patient safety (Islam et al., 2018). In terms of health promotion, statistical data and machine learning could be improved in the future to develop cost-efficient evaluation procedures and interventions (Michie et al., 2017). Such techniques could positively impact the population’s health by increasing the speed and reach of the health care system and making health care accessible for vulnerable populations. The knowledge of statistical data and its precise application to various cases lays a foundation for a successful work process and gives the individual an opportunity to show his expertise and improve his leadership skills.
Conclusion – Application of Statistics in Healthcare
Summing up, the statistical data, knowledge, application, and interpretation in health care are crucial. The data is used to provide quality health care services and to ensure patients’ safety. Statistical data could be collected in various forms, from direct interviews with the patients to fitness trackers. To provide effective use of patient data, health care organizations might introduce electronic systems that include machine learning. Machine learning could be used in many ways, from predicting surviving chances and making a diagnosis in a shorter amount of time to improving the quality of simulation exercises for the staff. Statistical application to health care implies that none of the vital information would be dismissed from the case. The basic knowledge of statistical data helps nurses when making decisions on treatment procedures based on the previous data on effectiveness.
In conclusion, this essay explored the topic of statistical application in the health care field and discussed its significance and how it is utilized in daily hospital work. It should be noted that the recent movement towards the application of statistical data is only possible because of a large amount of collected and organized data from the last decades. Further data collection would only improve the situation by making the evaluation and intervention processes faster, more precise, and more accessible to all layers of the population.
Beam A.L., & Kohane, I.S. (2018). Big data and machine learning in health care. Journal of American Medical Association. 319(13), 1317–1318.
Islam, M., Hasan, M., Wang, X., Germack, H., & Noor-E-Alam, M. (2018). A systematic review on healthcare analytics: Application and theoretical perspective of data Mining. Healthcare , 6 (2), 54.
Michie, S., Yardley, L., West, R., Patrick, K., & Greaves, F. (2017). Developing and evaluating digital interventions to promote behavior change in health and health care: Recommendations resulting from an international workshop. Journal of Medical Internet Research 19 (6), e232.
Cite this paper
Select style
- Chicago (A-D)
- Chicago (N-B)
NursingBird. (2023, July 31). Application of Statistics in Health Care – Importance & Examples of Use. https://nursingbird.com/application-of-statistics-in-health-care-essay-examples/
"Application of Statistics in Health Care – Importance & Examples of Use." NursingBird , 31 July 2023, nursingbird.com/application-of-statistics-in-health-care-essay-examples/.
NursingBird . (2023) 'Application of Statistics in Health Care – Importance & Examples of Use'. 31 July.
NursingBird . 2023. "Application of Statistics in Health Care – Importance & Examples of Use." July 31, 2023. https://nursingbird.com/application-of-statistics-in-health-care-essay-examples/.
1. NursingBird . "Application of Statistics in Health Care – Importance & Examples of Use." July 31, 2023. https://nursingbird.com/application-of-statistics-in-health-care-essay-examples/.
Bibliography
NursingBird . "Application of Statistics in Health Care – Importance & Examples of Use." July 31, 2023. https://nursingbird.com/application-of-statistics-in-health-care-essay-examples/.
- Desert Parkway Behavioral Healthcare Hospital: Data Analysis
- Healthcare Policy Analysis and Nurse Staffing Standards
- Servant Leadership in Health Care Setting
- Addressing the Issue of a Chemically Impaired Nurse
- Health Impacts of Residential School System on Indigenous People
- ICARE in the ICU Relations
- Routes of Administration Overview
- Dynamic Perspectives on Care among African-Americans
- Mastering Change in Jönköping County Council, Sweden
- The Complexity Involving Medicare Different Rate Reimbursement for NPs and MDs
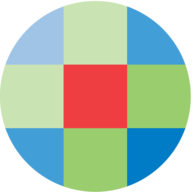
- Subscribe to journal Subscribe
- Get new issue alerts Get alerts
Secondary Logo
Journal logo.
Colleague's E-mail is Invalid
Your message has been successfully sent to your colleague.
Save my selection
Statistics in Nursing Research
Hayat, Matthew J. PhD
Assistant Professor and Biostatistician
College of Nursing, Rutgers University
Newark, New Jersey
[email protected]
The author has no funding or conflicts of interest to disclose.
Welcome to this focused issue on statistics in nursing research! Nursing Research put out a special call for papers on this topic in 2011. The response was significant and included 22 submitted manuscripts. Each submission went through a rigorous peer review process that included multiple statistical reviewers. This special issue presents 10 (of 11) accepted manuscripts. The articles cover a broad spectrum of statistical topics and represent recent advances in several important areas of applied statistics, including measurement, multilevel modeling, statistical inference, and causal inference.
Four articles focus on statistical considerations in instrument development and measurement. Stump et al. and Vessey et al. outline advantages to item response theory otherwise missed in classic testing theory. Sousa et al. and Pawlowitz et al. address the growing need in nursing science to compare psychometric instruments. The use of Bayesian statistical methods continues to grow by leaps and bounds in the statistics literature; it is fantastic to see Pawlowitz et al. apply this inference paradigm to a nursing science application. Analysis of correlated data is a hot topic in the nursing sciences, including topics such as the individual health trajectory, multilevel and hierarchical modeling, longitudinal data analysis, and latent variable modeling. Four articles touch on various aspects of these advanced statistical techniques. Hayat and Hedlin provide a nontechnical overview of this topic. Son et al. present an exciting approach for handling missing data using pattern mixture models in SPSS. Schmiege et al. give examples of research questions and corresponding types of latent variable and mixture models that can be used to address them. Eckardt as well as Coffman and Kugler each present methods for making causal inferences using propensity scoring. This is a promising area in the health sciences, as it enables a researcher to make causal statements in situations wherein it was not previously possible. Saylor et al. offer helpful insights into using SPSS to analyze large national databases with sample data obtained with complex sampling techniques.
Let’s consider these topics more generally in the context of science. The lifeline of a scientific investigation begins with a research question. A study is then designed to provide an answer. Rigorous science necessitates careful consideration of statistical considerations in the design of a research study to ensure an adequate answer. The natural next step is to conceive of measurable outcomes and accompanying instrumentation. An abundance of statistical considerations arise in the assessment of instrumentation or measurement. Finally, once data collection is complete, statistical analysis can be performed. The lifeline of scientific investigation is sequential and cumulative. For example, if the research question is poorly formulated, the steps to follow cannot compensate. Similarly, if the instrumentation is poor and does not adequately measure the outcome of interest, a sophisticated data analysis will be of little value.
As the nurse scientist progresses through this cumulative process, it is essential to develop and cultivate a statistical mind-set in the planning stages of research. This needed statistical mind-set goes well beyond considerations of data analysis or thinking of statistics as a tool. It is not enough to know which statistical test to use. Statistical thinking and reasoning is needed. As a statistician in a nursing college and collaborating with nurse scientists, I tell my colleagues to “come early, come often.” Always weary of the garbage in, garbage out principle, my contribution is most significant in the planning stages of research. There is little I am able to do if a research design is poor or instrumentation is inadequate.
Statistical training to develop a statistical mind-set is essential for the nurse scientist. Much can be learned about statistical training from a relatively new subfield of statistics referred to as statistics education research . The need for change in the way statistics is taught and thought about has been long overdue. For example, a study by Hogg (1991) found “Students often consider statistics as the ‘worst’ course they take while in college.” Statistical training for the nurse scientist can and should be fun and interesting. Recent research suggests that before delving into educating students about statistical methods, statistical literacy, thinking, and reasoning training is needed. In fact, studies have shown that a knowledge base in statistical literacy, reasoning, and thinking is needed for understanding published research ( delMas, Garfield, Ooms, & Chance, 2007 ).
I am happy to share news of recent efforts by statisticians to share nursing science with members of the statistics community. “Statistics in Nursing Research” was the theme of a contributed topic session and roundtable discussion at the 2011 Joint Statistical Meetings ( JSM, 2011 ; http://www.amstat.org/meetings/jsm/2011 ). The Joint Statistical Meetings is the largest gathering of statisticians held in North America. It is held jointly with the American Statistical Association and several international organizations. The meeting was attended by more than 5,000 statisticians. A group of statisticians from nursing schools participated in the conference and contributed to these sessions. In our focused sessions on “Statistics in Nursing Research,” speakers gave talks on statistical applications of multilevel modeling in the nursing sciences, analyzing longitudinal data in nursing research, and experiences and lessons learned in collaborating with nurse scientists. Roundtable discussion included topics related to (a) a faculty appointment as a statistician in a nursing school, (b) promotion, (c) statistics education for nursing students, (d) use of statistical software, (e) statistical consulting, and (f) the interesting multitude of roles for a statistician in an interdisciplinary setting, such as consulting, teaching, research, and service. It was a fun and fascinating experience to connect with other statisticians also collaborating with nurse scientists. There was much interest in continued discussion. We have a contributed topic session, panel discussion, and roundtable discussion on the topic of the use of statistics in the nursing sciences scheduled for the 2012 JSM ( http://www.amstat.org/meetings/jsm/2012 ).
Matthew J. Hayat, PhD
- Cited Here |
- + Favorites
- View in Gallery
Readers Of this Article Also Read
The development and testing of the nursing teamwork survey, effects of caring, measurement, and time on miscarriage impact and women's..., empirical development of a middle range theory of caring, towards development of nursing practice theory.
Statistics for Nursing Research Coursework
- To find inspiration for your paper and overcome writer’s block
- As a source of information (ensure proper referencing)
- As a template for you assignment
Introduction
The mean age of the sample data is 56.5 years. This implies that the arithmetic mean (average) across all the age-related observations in the sample data provided in the book is 56.5 years. The mean age of 56.5 years shows that the age values of the 10 patients are not skewed towards one end given that the minimum age is 35 years and the maximum age is 86 years.
Question 2: Percentage of Patients who Never Used Tobacco
From the analysis, it is clear that only 2 (20%) of the patients in the provided sample data never used tobacco. It is important to note that the value “N” represents those who never used tobacco in the sample data, while “F” denotes former use of tobacco and “C” stands for current use of tobacco by the patients.
Question 3: Standard Deviation for Age
The standard deviation for age is 19.25992. Since the standard deviation denotes a summary measure of the differences of each observation from the mean value, the large standard deviation demonstrated in the table can only imply that the age values in the provided data set are farther away from the mean age of 56.5 years, on average (Rumsey, 2016).
Question 4: Outliers among the Values of Age
An outlier, according to Grove and Cipher (2017), “is a value in a sample data set that is unusually low or unusually high in the context of the rest of the sample data” (p. 303). As shown from the analysis, the lowest age value in the dataset is 35 years and the highest is 86 years. However, all the other age values are evenly distributed between the lowest age value and the highest age value, meaning that there are no outliers or extreme values among the values of age. Additionally, there are no data points falling away as the extremes in the histogram (Knapp, 2017), meaning that the data set has no outliers among the values of age. Lastly, the SPSS Boxplot does not identify any outliers (often displayed as little circles with the ID number of the patient attached) (Rumsey, 2016).
Question 5: Range of Age Values
The range of age values is 51.00 years. Since the range denotes a measure of the spread of a particular variable (Grove & Cipher, 2017), the range value of 51 years is equal to the difference between the largest age value (86 years) and the smallest age value (35 years).
Question 6: Infliximab
From the analysis, it is clear that 6 (60%) of the patients in the sample data set had been taking Infliximab.
Question 7: Rheumatoid Arthritis
Half of the patients (50.0%) had a primary diagnosis for rheumatoid arthritis as the value “5” represents rheumatoid arthritis. It is important to note that the value of “5” was used to symbolize rheumatoid arthritis when entering data into the SPSS program.
Question 8: Irritable Bowel Syndrome
As demonstrated in the SPSS output table below, 3 (30%) of the patients had a primary diagnosis for irritable bowel syndrome as the value “3” represents irritable bowel syndrome. It is important to note that the value of “3” was used to symbolize irritable bowel syndrome when entering data into the SPSS program.
Question 9: Confidence Interval for Age
The 95% confidence interval for age is 42.7223 (lower bound) and 70.2777 (upper bound). This means that the lower (95%) confidence limit for the mean age is 42.7223 years, while the upper (95%) confidence limit for the mean age is 70.2777 years. Overall, it should be noted that “statisticians use a confidence interval to describe the amount of uncertainty associated with a sample estimate of a population parameter” (Knapp, 2017, p. 56).
Question 10: Psoriatic Arthritis
From the analysis, it is clear that only 1 (10.0%) of the patients had a primary diagnosis for psoriatic arthritis. The value “4” represents psoriatic arthritis. It is important to note that the value of “4” was used to symbolize psoriatic arthritis when entering data into the SPSS program.
Grove, S.K., & Cipher, D.J. (2017). Statistics for nursing research: A workbook for evidence-based practice (2nd ed.). St. Louis, MI: Elsevier.
Knapp, H. (2017). Practical statistics for nursing using SPSS . Thousand Oaks, CA: Sage Publishing.
Rumsey, D.J. (2016). Statistics for dummies (2nd ed.). New York, NY: For Dummies Publishing.
- Differences in Everyday Timings for Reaching College
- The Use of Graphics and Statistics to Misrepresent Data
- Rheumatoid Arthritis and Age Factor of Autoimmunity
- The Rheumatoid Arthritis Analysis
- Pharmacotherapy for Rheumatoid Arthritis
- Local Talent vs. Expat Talent in UAE Private Sector
- Vacuum Cleaner Design Study
- Using Minitab Descriptive Statistics
- Non-Parametric and Parametric Tests
- Determinant Factors for the Longevity of Humans Through Statistical Analysis
- Chicago (A-D)
- Chicago (N-B)
IvyPanda. (2022, June 4). Statistics for Nursing Research. https://ivypanda.com/essays/statistics-for-nursing-research/
"Statistics for Nursing Research." IvyPanda , 4 June 2022, ivypanda.com/essays/statistics-for-nursing-research/.
IvyPanda . (2022) 'Statistics for Nursing Research'. 4 June.
IvyPanda . 2022. "Statistics for Nursing Research." June 4, 2022. https://ivypanda.com/essays/statistics-for-nursing-research/.
1. IvyPanda . "Statistics for Nursing Research." June 4, 2022. https://ivypanda.com/essays/statistics-for-nursing-research/.
Bibliography
IvyPanda . "Statistics for Nursing Research." June 4, 2022. https://ivypanda.com/essays/statistics-for-nursing-research/.

An official website of the United States government
The .gov means it’s official. Federal government websites often end in .gov or .mil. Before sharing sensitive information, make sure you’re on a federal government site.
The site is secure. The https:// ensures that you are connecting to the official website and that any information you provide is encrypted and transmitted securely.
- Publications
- Account settings
Preview improvements coming to the PMC website in October 2024. Learn More or Try it out now .
- Advanced Search
- Journal List
- HHS Author Manuscripts

Increasing Quantitative Literacy in Nursing: A Joint Nursing-Statistician Perspective
Krista schroeder.
a Assistant Professor of Nursing, Temple University College of Public Health, 3307 North Broad Street, Philadelphia PA 19140, USA
Levent DUMENCI
b Professor of Epidemiology and Biostatistics, Temple University College of Public Health, Philadelphia PA 19122, USA
David B. SARWER
c Associate Dean for Research, Director - Center for Obesity Research and Education, Temple University College of Public Health, Philadelphia PA 11940, USA
David C. WHEELER
d Associate Professor, Virginia Commonwealth University School of Medicine, Department of Biostatistics, Richmond VA 23298, USA
Matthew J. HAYAT
e Professor of Biostatistics, School of Public Health & Byrdine F. Lewis College of Nursing and Health Professions at Georgia State University, Atlanta GA 30302, USA
Strong quantitative literacy is necessary to fulfill nurses’ professional responsibilities across education levels, roles, and settings. Evidence-based practice and systems improvement are not possible if nurses do not understand the statistics employed in generating evidence. Statistics is the language of science and rigorous nursing science cannot exist without a clear understanding of statistical methods. Increasing availability and complexity of medical and public health data and a growing focus on population health necessitate increasingly sophisticated quantitative literacy in nursing practice, leadership, and science ( Hayat, Kim, Schwartz, & Jiroutek, 2021 ; Hayat, Schmiege, & Cook, 2014 ). Without strong quantitative knowledge, the nursing profession will lose opportunities to lead evidence-driven, population-focused efforts for health improvement.
Serious limitations in both knowledge and application of statistics have been documented in nursing pedagogy, scholarship, and research for decades ( Anthony, 1996 ; Gaskin & Happell, 2014 ; Hayat, Higgins, Schwartz, & Staggs, 2015 ; Hayat et al., 2021 ; Hayat et al., 2014 ). Prior work calling for greater quantitative literacy in nursing has been solely or primarily statistician-led, leaving an opportunity and responsibility for nurses to contribute. Without the voice of nursing, efforts to improve quantitative literacy within the profession will lack nursing insight and perspective. In this commentary we provide guidance for nurses’ engagement with quantitative methods and offer suggestions to increase quantitative literacy in nursing across education levels, roles, and settings.
Be Aware of What You Do and Don’t Know
For nurses, awareness of one’s level of statistical knowledge can foster more effective communication with statisticians and consumers of nursing scholarship and avoid analytic errors ground in lack of statistical knowledge. Statistics is a discipline – not a toolbox; statistics is not simply about choosing the right analytic approach, but about a start-to-finish approach to project planning, data collection, appropriate analysis (including confirming underlying statistical assumptions and conducting sensitivity analyses), and accurately and comprehensively understanding and presenting results to a range of stakeholders with varying levels of statistical knowledge. Importantly, levels of required statistical knowledge varies by nursing role – BSN-prepared clinicians focus on evidence-based practice, MSN- and DNP-prepared nurse practitioners focuses on quality improvement and research translation to systems and practice, and PhD-prepared nurse scientists focus on creation of generalizable knowledge ( Hayat et al., 2014 ). Thus, while BSN-, MSN-, and DNP-prepared nurses may focus on quantitative literacy, PhD-prepared nurses may recognize larger gaps in their required knowledge and focus on gaining statistical expertise required to conduct high quality nursing research.
Know How to Find Statistical Help
Many nurses – particularly those working primarily in clinical practice, in a small organization, or in a setting without formal academic-practice partnerships – may be unsure how to find statistical assistance. Often, nurses are not taught how to collaborate with statisticians ( Hayat et al., 2015 ). A first step entails deciding from whom statistical help is needed. A graduate student in statistics, MS-prepared statistician, and PhD-prepared statistician will bring different expertise, but all may be appropriate collaborators depending on need. A nurse scientist conducting research to develop new knowledge likely requires different expertise than a hospital nursing unit manager planning a quality improvement project. Statistical collaborators are often available via academic institutions (schools of nursing but also schools of public health or medicine). Nursing-statistician collaboration typically necessitates deeper partnership than simply confirming which statistical test should be used, as statisticians can help with the entire project planning process ( Hayat et al., 2015 ). Nursing collaborators should be aware that statistical collaboration may entail cost such as hourly fees or coverage of salary/effort. Alternatively, statistical support services may be provided by a nursing school or college, such as via consulting labs, that do not require funding or provide short term consultation as needed.
When seeking a statistical collaborator, it is important to be aware that statisticians have different focuses and areas of expertise. Simply finding “a statistician” may be too broadly defined and thus an ineffective approach. For example, the statistician who helps with instrument development may not be the same statistician who helps plan a randomized controlled trial nor the same statistician who helps analyze large, multi-level data from an electronic medical record. Attention to statisticians’ areas of expertise is important to finding the right collaborator.
For PhD-prepared Nurses, Seek Challenging Learning Opportunities
Nurses should embrace challenge when learning about statistics. For PhD-prepared nurses, gaining statistical expertise through summer intensive or short courses, formal university coursework, or career development awards is often beneficial. Particularly for nurse scientists whose work entails advanced quantitative approaches, such additional training is likely a necessity. PhD-prepared nurses should also consider challenging themselves to learn analytic tools beyond menu-driven commercial software (e.g., SPSS) ( Hayat et al., 2014 ). Code-driven and free-of-charge statistical computing tools allow for replicability, transparency, and documentation of analytic work. R is a tool that may be of particular interest, given its open structure, large and active user-driven community, and availability of numerous flexible user-provided packages. R is also a useful tool for working with spatial data, which is relevant for nurses who are interested in social or environmental determinants of health, such as neighborhood poverty or greenspace access. Thus, while learning tools beyond menu-driven software may initially feel challenging, doing so can contribute meaningfully to one’s statistical skillset.
Focus on Quantitative Literacy Rather Than Statistical Expertise
For most nurses, the goal should be quantitative literacy ( Hayat et al., 2015 ; Hayat et al., 2014 ). Nurses bring important content expertise coupled with a wealth of relevant clinical experience that can bring data analytic strategies alive for a multidisciplinary audience in a presentation or manuscript. A statistician would not take one or two courses in “nursing” and expect to care for patients. Similarly, a nurse should not take one or two biostatistics courses and aim to plan, execute, and interpret one’s own data analysis. Nurses can approach statistical collaboration with respect for the content knowledge they bring as a nurse, rather than an insecurity about the statistical expertise they lack. Through effective collaboration and a focus on strong quantitative literacy, nurses can dispel negative stereotypes about nurses not being “good at” statistics.

Advance Efforts to Increase Quantitative Literacy in Nursing
Given documented gaps in nursing knowledge, strategies for increasing quantitative literacy must be considered. Quantitative and qualitative assessments of nurses’ statistical learning needs could inform translation of the robust statistics education literature into nursing training. A nursing-focused addendum to the Guidelines for Assessment and Instruction in Statistics Education could inform nursing education, as could increased guidance on nursing education statistical competencies from accrediting bodies. In addition, formalizing processes and enforcing rigorous guidelines for manuscripts’ statistical methods sections in nursing journals could increase rigor within the nursing literature ( Hayat et al., 2015 ; Hayat et al., 2021 ). Further, efforts to increase the pipeline of individuals well-prepared to serve as statisticians in schools of nursing could benefit academic nursing. Faculty who hold joint appointment in nursing and statistics and have formal education and training in both fields may be optimal, and they would have both scholarly authority in nursing and statistics and the ability to communicate effectively with nursing students. Joint graduate degree programs or minors – approaches used by many other disciplines at large research universities – can increase the pipeline of statisticians prepared in this manner and well-suited to serve in schools of nursing.
Conclusions
There is a ripe opportunity for increased nursing leadership to improve quantitative literacy in nursing. An active collaboration of nursing and statistical thought leaders can chart the path forward. When armed with appropriate statistical knowledge, nurses can play a unique role in using data to promote health and prevent disease among individuals, communities, and populations.
This research was funded by the Eunice Kennedy Shriver National Institute of Child Health and Human Development (K23 HD101554; PI: Schroeder) of the National Institutes of Health (NIH). Dr. Sarwer’s work was supported by grant funding from the National Institutes of Health (National Institute for Diabetes, Digestive, and Kidney Disease R01 DK108628 and National Institute of Dental and Craniofacial Research R01 DE026603) as well as PA CURE Funds from the Commonwealth of Pennsylvania. The content is solely the responsibility of the authors and does not necessarily represent the views of the funder. The funder had no role in the development or preparation of this manuscript.
Conflict of Interest Statement: Krista Schroeder, Levent Dumenci, David C. Wheeler, and Matthew J. Hayat declare that they have no conflict of interest. David Sarwer discloses consulting relationships with Ethicon and NovoNordisk.
- Anthony D (1996). A review of statistical methods in the Journal of Advanced Nursing. Journal of Advanced Nursing , 24 ( 5 ), 1089–1094. [ PubMed ] [ Google Scholar ]
- Gaskin CJ, & Happell B (2014). Power, effects, confidence, and significance: An investigation of statistical practices in nursing research. International Journal of Nursing Studies , 51 ( 5 ), 795–806. [ PubMed ] [ Google Scholar ]
- Hayat MJ, Higgins M, Schwartz TA, & Staggs VS (2015). Statistical challenges in nursing education and research: An expert panel consensus. Nurse Educator , 40 ( 1 ), 21–25. [ PubMed ] [ Google Scholar ]
- Hayat MJ, Kim M, Schwartz TA, & Jiroutek MR (2021). A study of statistics knowledge among nurse faculty in schools with research doctorate programs. Nursing Outlook , 69 ( 2 ), 228–233. [ PubMed ] [ Google Scholar ]
- Hayat MJ, Schmiege SJ, & Cook PF (2014). Perspectives on Statistics Education: Observations From Statistical Consulting in an Academic Nursing Environment. Journal of Nursing Education , 53 ( 4 ), 185–191. [ PubMed ] [ Google Scholar ]
Nursing Essay Examples

Nursing Essay Examples That Will Help You Write a Stellar Paper
Published on: May 6, 2023
Last updated on: Jan 29, 2024
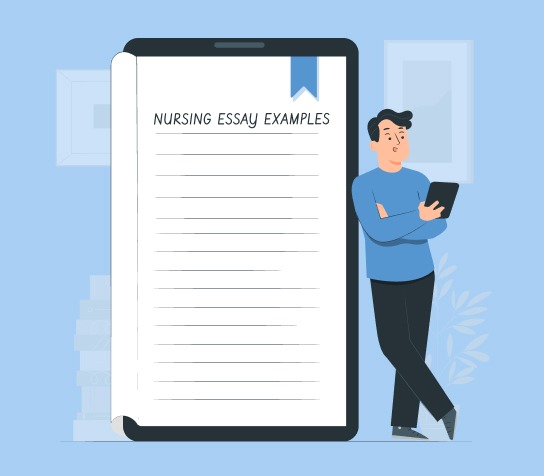
Share this article
Many nursing students struggle with writing effective nursing essays, which are an essential part of their education and professional development.
Poor essay writing skills can lead to low grades and an inability to effectively communicate important information.
This blog provides a comprehensive guide to writing nursing essays with examples and tips for effective writing. Whether you are a nursing student or a professional looking to improve your writing skills, this blog has something for you.
By following the tips and examples provided, you can write compelling nursing essays that showcase your dedication to the field.
Let’s get started.
On This Page On This Page -->
What is a Nursing Essay?
A nursing essay is a type of academic writing that aims to explore a particular topic related to nursing. It also presents a clear and concise argument or viewpoint supported by evidence.
Nursing essays can take many forms, including:
- Descriptive essays
- Reflective essays
- Analytical essays
- Persuasive essays
What is the Importance of the Nursing Essay?
Nursing essays are important for several reasons. First, they help nursing students develop critical thinking skills by requiring them to analyze and evaluate information.
Second, they help students develop research skills by requiring them to locate and use credible sources to support their arguments.
Third, nursing essays help students develop communication skills by requiring them to present their ideas clearly and concisely in writing. Finally, nursing essays are important for nursing education because they prepare students for the types of writing.
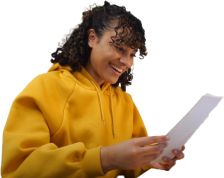
Paper Due? Why Suffer? That's our Job!
To help students better understand how to write nursing essays, it can be helpful to review examples.
Below are some examples of nursing essays.
Nursing School Essay Examples
College Nursing Essay Examples
Graduate Nursing Essay Examples
Nursing Scholarship Essay Examples
Nursing Essay Conclusion Examples
Nursing Essay Examples of Different Fields
Nursing is a diverse field with many different specialties and areas of focus. As a result, nursing essays can take many different forms and cover a wide range of topics.
Given below are some examples of different types of nursing essays:
Personal Philosophy Of Nursing - Essay Examples
Cal State Fullerton Nursing Essay Examples
Evidence Based Practice Nursing In Medical Field - Essay Examples
Leadership In Nursing And Healthcare Professionals - Essay Examples
Principles Of Professional Practice Of Nursing Professionals And Pharmacists
If you're seeking additional examples of nursing essays, you're in luck!
Below are some more examples that can help you gain a better understanding of nursing essays:
Health Care And Reflective Models For Nursing - Essay Examples
History Of Nursing Essay Examples
Ethical Dilemma In Nurses Work - Essay Examples
Mental Health Nursing Essay Examples
Why I Want To Be A Nurse Essay
Working In A Team And Collaboration In Nursing
How to Write a Nursing Essay
Writing a nursing essay can seem daunting, but with the right approach, it can be a rewarding experience.
Here are the key steps involved in writing a nursing essay:
Understanding the Topic and Question
The first step in writing a nursing essay is to carefully read and understand the topic and question.
This will help you determine what information you need to research and include in your essay. Make sure you understand any key terms or concepts related to the topic. Consider different perspectives or viewpoints that may be relevant.
Researching the Topic
Once you have a clear understanding of the topic and question, it's time to research.
Start by gathering information from credible sources such as academic journals, textbooks, and government websites.
Consider both primary and secondary sources, and make sure to take detailed notes as you read.
Organizing and Outlining the Essay
Once you have completed your research, it's time to organize your ideas and create an outline for your essay.
Start by identifying the main points or arguments you want to make, and then organize them into a logical order that flows well.
Your outline should include an introduction, body paragraphs, and a conclusion.
Writing the Essay
With your outline in place, it's time to start writing your essay. Make sure to follow your outline closely, and use clear and concise language that effectively communicates your ideas.
Use evidence from your research to support your arguments, and cite your sources appropriately.
Editing and Revising the Essay
Once you have completed a first draft of your essay, take some time to edit and revise it. Look for any errors in grammar, spelling, or punctuation, and make sure your essay is well-organized and flows well.
Consider asking a peer or instructor to review your essay and provide feedback.
What To Include In Your Nursing Essay
When writing a nursing essay, there are several key elements that you should include. Here are some important things to keep in mind:
- Introduction
Your introduction should provide a brief overview of the topic and purpose of your essay. It should also include a clear thesis statement that presents your main argument or point of view.
- Background Information
Provide some background information on the topic to help the reader better understand the context of your essay. This can include relevant statistics, historical information, or other contextual details.
- Evidence and Examples
Use evidence and examples from your research to support your arguments and demonstrate your knowledge of the topic. Make sure to cite your sources appropriately and use a variety of sources to strengthen your argument.
- Analysis and Evaluation
Provide analysis and evaluation of the evidence and examples you've presented. This can include discussing strengths and weaknesses, comparing and contrasting different viewpoints, or offering your own perspective on the topic.
Your conclusion should summarize the main points of your essay and restate your thesis statement. It should also offer some final thoughts or suggestions for further research or action.
Nursing Essay Topic Ideas
Choosing a topic for your nursing essay can be challenging, but there are many areas in the field that you can explore. Here are some nursing essay topic ideas to consider:
- The role of technology in nursing practice
- The impact of cultural diversity on healthcare delivery
- Nursing leadership and management in healthcare organizations
- Ethical issues in nursing practice
- The importance of patient-centered care in nursing practice
- The impact of evidence-based practice on nursing care
- The role of nursing in promoting public health
- Nursing education and the importance of lifelong learning
- The impact of nursing shortages on healthcare delivery
- The importance of communication in nursing practice
These are just a few ideas to get you started. You can also explore other topics related to nursing that interest you or align with your academic or professional goals.
Remember to choose a topic that is relevant, interesting, and feasible to research and write about.
Tips for Writing an Effective Nursing Essay
Writing a successful nursing essay requires careful planning, research, and attention to detail. Here are some tips to help you write an effective nursing essay:
- Writing Concisely and Clearly
Nursing essays should be written in clear and concise language, avoiding unnecessary jargon or technical terms. Use simple language and short sentences to help ensure that your ideas are communicated clearly and effectively.
- Stating a Clear Thesis Statement
Your thesis statement should clearly state your main argument and provide a roadmap for the rest of your essay. It should be clear, concise, and located at the end of your introduction.
- Using Proper Citation and Referencing
Citing and referencing your sources is crucial in any academic writing, including nursing essays. Make sure to use proper citation and referencing styles, such as APA or MLA. Include a reference list or bibliography at the end of your essay.
- Seeking Feedback and Revising
Before submitting your nursing essay, seek feedback from peers, professors, or writing tutors. Use their feedback to revise and improve your essay. Make sure that it is well-structured, coherent, and effectively communicates your point of view.
By following these tips, you can write a nursing essay that demonstrates your knowledge and skills in the field.
In conclusion, writing a successful nursing essay requires careful planning, research, and attention to detail.
To showcase your knowledge in the field of nursing, it is important to have a clear understanding of the topic at hand. When writing your nursing essay, be sure to include relevant examples, incorporate current research, and use proper citation and referencing.
And remember , seeking feedback and revising your essay is key to ensuring that it effectively communicates your ideas and arguments.
If you need help with your nursing essay or any other type of academic writing, consider using our AI essay writer .
Our nursing essay writing service can provide personalized support to help you succeed in your academic goals.
So, why wait? Contact us to get college essay writing help today!
Cathy A. (Literature)
For more than five years now, Cathy has been one of our most hardworking authors on the platform. With a Masters degree in mass communication, she knows the ins and outs of professional writing. Clients often leave her glowing reviews for being an amazing writer who takes her work very seriously.
Paper Due? Why Suffer? That’s our Job!
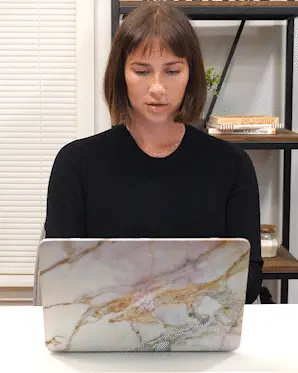
Legal & Policies
- Privacy Policy
- Cookies Policy
- Terms of Use
- Refunds & Cancellations
- Our Writers
- Success Stories
- Our Guarantees
- Affiliate Program
- Referral Program
- AI Essay Writer
Disclaimer: All client orders are completed by our team of highly qualified human writers. The essays and papers provided by us are not to be used for submission but rather as learning models only.

Describe the application of statistics in health care essay HLT-362V Solution
- Dr. Rachel Andel
- October 27, 2022
- Nursing Paper Examples
Describe the application of statistics in health care. Specifically discuss its significance to quality, safety, health promotion, and leadership. Consider your organization or specialty area and how you utilize statistical knowledge in patient fall reduction. Discuss how you obtain statistical data, how statistical knowledge is used in day-to-day operations and how you apply it or use it in decision making.
Are you looking for answers to a similar assignment? Nursingstudy.org has the top and most qualified writers to help with any of your assignments. All you need to do is place an order with us. (Describe the application of statistics in health care HLT-362V Solution)
HLT-362V Application of Statistics in Health Care –
Statistical application and the interpretation of data is important in health care. Review the statistical concepts covered in this topic. In a 750-1,000 word paper, discuss the significance of statistical application in health care . Include the following:
- Describe the application of statistics in health care. Specifically discuss its significance to quality, safety, health promotion, and leadership. ( application of statistics in health care essay)
- Consider your organization or specialty area and how you utilize statistical knowledge in patient fall reduction. Discuss how you obtain statistical data, how statistical knowledge is used in day-to-day operations and how you apply it or use it in decision making. what is the importance of statistics in healthcare, historical application of statistics in healthcare, 10 uses of health statistics, importance of statistics in public health, statistics in healthcare examples, samples descriptive statistics summarize, statistics in healthcare
Three peer-reviewed, scholarly or professional references are required.
Prepare this assignment according to the guidelines found in the APA Style Guide
HLT-362V Application of Statistics in Health Care – Application of Statistics in Health Care
Describe the application of statistics in health care.
The healthcare industry has significantly adopted the use of statistics in their operations. Statistics is the field of science concerned with data management. The field entails gathering data about diverse populations and their manipulations to infer meaning. The data is then presented to diverse stakeholders in the form they understand better. Application of healthcare statistics is seen in the different significance it holds, as highlighted by its value to service quality, health promotion, safety, and good leadership.
Healthcare organizations have resorted to the use of statistics to enhance the quality of service delivery. The value of quality cannot be ignored in any organization. Quality in the healthcare industry can be looked at from different perspectives. However, the quality attribute is to attend to the patient’s treatment needs to meet their expectations. The fact that patients have diverse complications means they value quality in healthcare organizations attending to their needs conveniently. Healthcare organizations have taken advantage of statistics to improve the quality of services they offer (Pett, 2015).
One such aspect of service improvement is seen in the use of patients’ remarks on quality standards. Healthcare organizations provide patients with different platforms to give feedback on the services they received. Access to a vast pool of patient’s information on different services allows the organizations to pinpoint particular areas where service improvement is required. The information may be brought to light using various statistical tools such as percentages, frequencies, and means regarding a particular condition. Identification of the critical areas of concern allows healthcare organizations to improve on the weaknesses, thus leading to betters service delivery (National Center for Health Statistics, 2017). Therefore, healthcare statistics are illustrated to contribute to value enhancement by analyzing data to determine areas of weaknesses, thereby improving them.
Another application of healthcare statistics is a safety enhancement. Safety is considered a vital aspect of any treatment procedure. The sole purpose of individuals seeking medical help at healthcare organizations is to improve their health conditions. There is no particular time when a patient expects their health condition to worsen after receiving treatment. Attainment of better healthcare means that medical practitioners and organizations provide patients with services that do not pose any risks. Healthcare statistics allows the medical team to discover safe treatment procedures.
Data acquisition and research activities also offer avenues for treatments on evidence-based practice. Knowledge of the risks is attained from scholarly sources such as statistical findings of the condition a patient portrays and risks associated with different treatments. Identification of the patient’s treatment risks allows the organization to pursue alternative treatments that do not interfere with their wellbeing. The promotion of safety in the healthcare sector is also made possible by identifying and lowering medical error cases. Healthcare organizations can rely on data about diverse medical errors in the organization. Determinations of the errors and their causes guide the organization to enact appropriate actions to reduce such cases, thereby leading to the promotion of safety standards.
Additionally, healthcare statistics can be used in steering the decision-making process in organizations leading to better leaders. The ability to make meaningful decisions relies on quantity and quality information (Amante et al. 2015). The quantity aspect requires the availability of adequate information that can make the findings significant. Likewise, the information’s credibility is depicted in its reliability in portraying the data’s actual image. Access to healthcare statistics enhances the leadership skills of hospital managers. Analysis of the data allows the managers to pinpoint diverse aspects of the organization’s performance. For instance, the manager can identify challenges in particular units after the interpretation of statistical data. The manager will then respond by putting in place the appropriate measures to solve the challenges through good decision making. Healthcare statistics, therefore, contribute to better leaders by enhancing their decision-making capabilities.
Healthcare statistics are also vital in promoting health conditions. The statistics inform healthcare stakeholders of various conditions, thereby facilitating the undertaking of corrective measures. For instance, healthcare practitioners rely on statistical figures to determine whether a particular health condition qualifies to be considered a pandemic. Health officials look at different aspects, such as the spread of the health condition, the affected population, and the mortality rate. Understanding the severity of the conditions prompting the respective agencies to put in place better response procedures. Likewise, healthcare statistics are used by different stakeholders to identify diverse aspects of clinical health that will allow better service delivery. An example is depicted by the government determining statistics about common diseases and allocating the necessary resources to improve service delivery for the identified positions.
A good illustration of the use of healthcare statistics in my organization is in preventing patients’ falls. Data on patient’s falls can be accessed from the electronic health record systems. Data adequacy will be provided by the coverage of a considerable period; for instance, the statistics will review patient falls for five years. Analyzing the data will provide the organization with information on the areas to improve on regarding the falls. For instance, the managers will identify the types of falls, prevalent fall areas, and factors leading to the falls. Therefore, the information will guide the organization in coming up with different ways to minimize the different causes of falls by putting in place ideal preventive measures. The daily use of statistics is seen in the continuous recording of diverse patient data. The collected data which form part of the daily routines is eventually used to analyze data in the long run.
In conclusion, healthcare statistics have been highlighted as significant for medical organizations. The statistics have been highlighted to provide healthcare organizations with diverse information to guide their decision-making. Healthcare statistics have been depicted to enhance service value, promote health, safety, and develop better leaders through effective decision-making.
Are you looking for answers to a similar assignment? Nursingstudy.org has the top and most qualified writers to help with any of your assignments. All you need to do is place an order with us. (Describe the application of statistics in health care HLT-362V Solution)

Amante, D. J., Hogan, T. P., Pagoto, S. L., English, T. M., & Lapane, K. L. (2015). Access to care and use of the Internet to search for health information: results from the US National Health Interview Survey. Journal of medical Internet research, 17(4), e106.
National Center for Health Statistics. (2017). Health, United States, 2016, with chartbook on long-term trends in health (No. 2017). Government Printing Office.
Pett, M. A. (2015). Nonparametric statistics for health care research: Statistics for small samples and unusual distributions. Sage Publications.
Community Health Promotion Essay Examples
Discussion: NURSFPX4060 Capella University Health Promotion Plan and Best Practices PPT Health Promotion Plan | |
Health Promotion Plan Presentation | |
Identify interdisciplinary health professionals important to include in the health promotion. What is their ? Why is their involvement significant? | |
PURPOSE To apply concepts you have learned about health promotion concepts and strategies, enhance your written communication skills, and demonstrate a beginning understanding of cultural competency. | |
Pender Health Promotion Model |
Working On an Assignment With Similar Concepts Or Instructions?
A Page will cost you $12, however, this varies with your deadline.
We have a team of expert nursing writers ready to help with your nursing assignments. They will save you time, and improve your grades.
Whatever your goals are, expect plagiarism-free works, on-time delivery, and 24/7 support from us.
Here is your 15% off to get started. Simply:
- Place your order ( Place Order )
- Click on Enter Promo Code after adding your instructions
- Insert your code – Get20
All the Best,
Have a subject expert Write for You Now
Have a subject expert finish your paper for you, edit my paper for me, have an expert write your dissertation's chapter, what you'll learn.
- Nursing Essay Examples
Related Posts
- Personal Philosophy Statement Example for a PMHNP
- Leadership Strategies for Success-Nursing Paper Examples
- Applying the Scientific Method-Nursing Paper Examples
Important Links
Knowledge base, paper examples, nursing writing services.
Nursingstudy.org helps students cope with college assignments and write papers on various topics. We deal with academic writing, creative writing, and non-word assignments.
All the materials from our website should be used with proper references. All the work should be used per the appropriate policies and applicable laws.
Our samples and other types of content are meant for research and reference purposes only. We are strongly against plagiarism and academic dishonesty.
Phone: +1 628 261 0844
Mail: [email protected]
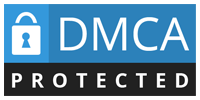
We Accept:

@2015-2024, Nursingstudy.org
24/7 writing help on your phone
To install StudyMoose App tap and then “Add to Home Screen”
Practical Application of Statistics in Nursing
Save to my list
Remove from my list

Uses of Statistics in Healthcare
Statistics used in the workplace, descriptive statistics, inferential statistics, four levels of measurement, advantages of accurate interpretation of statistical information.
- Levels of Measurement. (n.d.). Retrieved January 23, 2015, from http://onlinestatbook.com/2/introduction/levels_of_measurement.html
- Bennett, J. O., Briggs, W. L., ; Triola, M. F. (2009). Statistical Reasoning for Everyday Life (3rd ed.). Retrieved from The University of Phoenix eBook Collection Database
Practical Application of Statistics in Nursing. (2016, Sep 23). Retrieved from https://studymoose.com/practical-application-of-statistics-in-nursing-essay
"Practical Application of Statistics in Nursing." StudyMoose , 23 Sep 2016, https://studymoose.com/practical-application-of-statistics-in-nursing-essay
StudyMoose. (2016). Practical Application of Statistics in Nursing . [Online]. Available at: https://studymoose.com/practical-application-of-statistics-in-nursing-essay [Accessed: 7 Sep. 2024]
"Practical Application of Statistics in Nursing." StudyMoose, Sep 23, 2016. Accessed September 7, 2024. https://studymoose.com/practical-application-of-statistics-in-nursing-essay
"Practical Application of Statistics in Nursing," StudyMoose , 23-Sep-2016. [Online]. Available: https://studymoose.com/practical-application-of-statistics-in-nursing-essay. [Accessed: 7-Sep-2024]
StudyMoose. (2016). Practical Application of Statistics in Nursing . [Online]. Available at: https://studymoose.com/practical-application-of-statistics-in-nursing-essay [Accessed: 7-Sep-2024]
- Crafting Connections: Language Dynamics and Practical Application Pages: 4 (961 words)
- L.E.A.R.N Assignment - Role Development for Practical Nursing Pages: 3 (871 words)
- The Ways How to Become a Successful Practical Nursing Student Pages: 2 (533 words)
- The Application of Management Theories in the Field of Nursing Pages: 2 (362 words)
- Application of Theory: Patient-Centered Nursing Framework Pages: 4 (1199 words)
- The practical life area in a Montessori classroom Pages: 6 (1574 words)
- The principle underlying the Practical Life exercises Pages: 8 (2244 words)
- Personal & Practical Applications Pages: 11 (3066 words)
- Conservation of Momentum Practical Write Up Pages: 5 (1496 words)
- McClelland’s Three Manifest Needs Theory and Its Practical Implications Pages: 6 (1518 words)
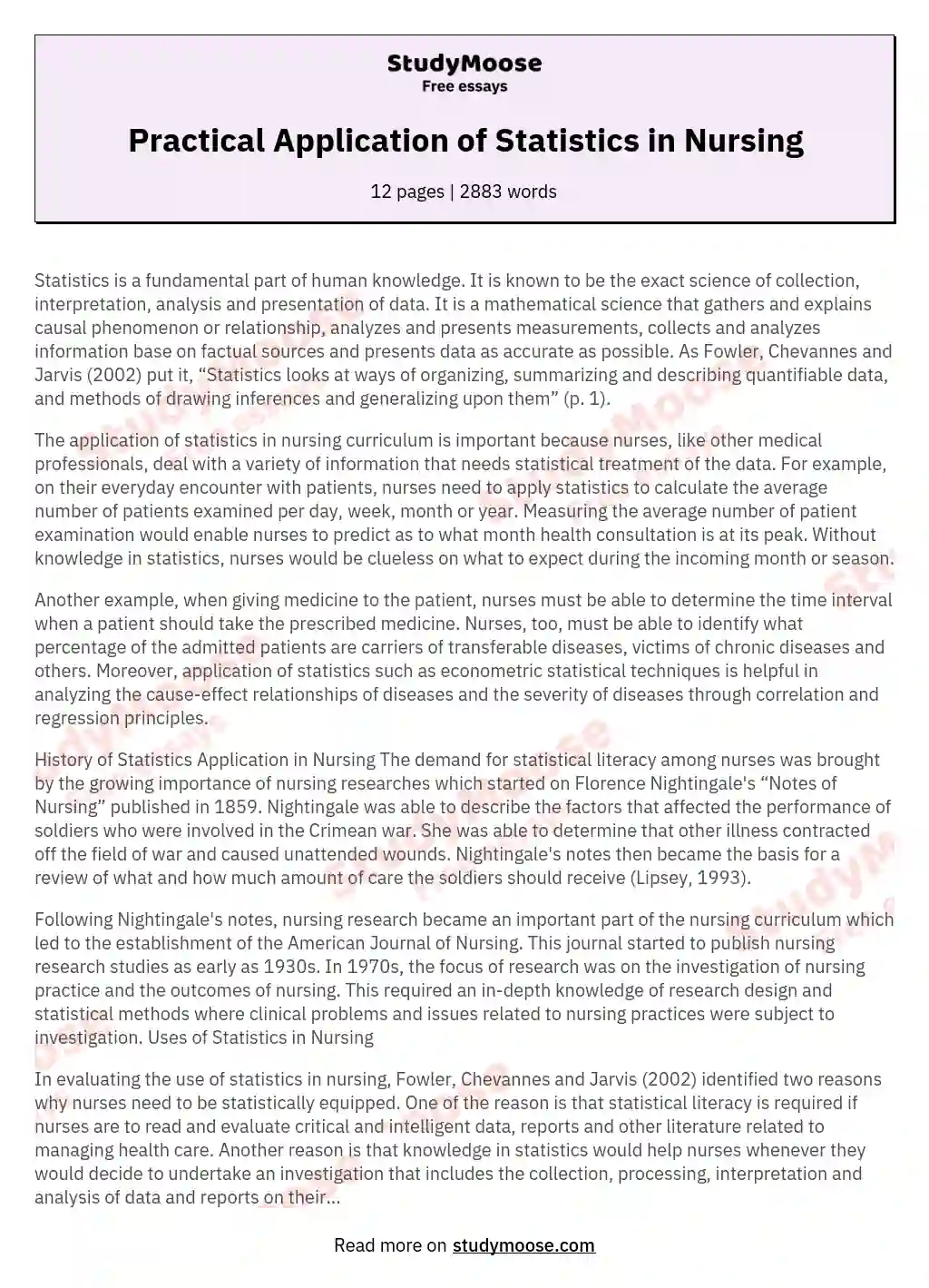
👋 Hi! I’m your smart assistant Amy!
Don’t know where to start? Type your requirements and I’ll connect you to an academic expert within 3 minutes.
Have a language expert improve your writing
Run a free plagiarism check in 10 minutes, generate accurate citations for free.
- Knowledge Base
The Beginner's Guide to Statistical Analysis | 5 Steps & Examples
Statistical analysis means investigating trends, patterns, and relationships using quantitative data . It is an important research tool used by scientists, governments, businesses, and other organizations.
To draw valid conclusions, statistical analysis requires careful planning from the very start of the research process . You need to specify your hypotheses and make decisions about your research design, sample size, and sampling procedure.
After collecting data from your sample, you can organize and summarize the data using descriptive statistics . Then, you can use inferential statistics to formally test hypotheses and make estimates about the population. Finally, you can interpret and generalize your findings.
This article is a practical introduction to statistical analysis for students and researchers. We’ll walk you through the steps using two research examples. The first investigates a potential cause-and-effect relationship, while the second investigates a potential correlation between variables.
Table of contents
Step 1: write your hypotheses and plan your research design, step 2: collect data from a sample, step 3: summarize your data with descriptive statistics, step 4: test hypotheses or make estimates with inferential statistics, step 5: interpret your results, other interesting articles.
To collect valid data for statistical analysis, you first need to specify your hypotheses and plan out your research design.
Writing statistical hypotheses
The goal of research is often to investigate a relationship between variables within a population . You start with a prediction, and use statistical analysis to test that prediction.
A statistical hypothesis is a formal way of writing a prediction about a population. Every research prediction is rephrased into null and alternative hypotheses that can be tested using sample data.
While the null hypothesis always predicts no effect or no relationship between variables, the alternative hypothesis states your research prediction of an effect or relationship.
- Null hypothesis: A 5-minute meditation exercise will have no effect on math test scores in teenagers.
- Alternative hypothesis: A 5-minute meditation exercise will improve math test scores in teenagers.
- Null hypothesis: Parental income and GPA have no relationship with each other in college students.
- Alternative hypothesis: Parental income and GPA are positively correlated in college students.
Planning your research design
A research design is your overall strategy for data collection and analysis. It determines the statistical tests you can use to test your hypothesis later on.
First, decide whether your research will use a descriptive, correlational, or experimental design. Experiments directly influence variables, whereas descriptive and correlational studies only measure variables.
- In an experimental design , you can assess a cause-and-effect relationship (e.g., the effect of meditation on test scores) using statistical tests of comparison or regression.
- In a correlational design , you can explore relationships between variables (e.g., parental income and GPA) without any assumption of causality using correlation coefficients and significance tests.
- In a descriptive design , you can study the characteristics of a population or phenomenon (e.g., the prevalence of anxiety in U.S. college students) using statistical tests to draw inferences from sample data.
Your research design also concerns whether you’ll compare participants at the group level or individual level, or both.
- In a between-subjects design , you compare the group-level outcomes of participants who have been exposed to different treatments (e.g., those who performed a meditation exercise vs those who didn’t).
- In a within-subjects design , you compare repeated measures from participants who have participated in all treatments of a study (e.g., scores from before and after performing a meditation exercise).
- In a mixed (factorial) design , one variable is altered between subjects and another is altered within subjects (e.g., pretest and posttest scores from participants who either did or didn’t do a meditation exercise).
- Experimental
- Correlational
First, you’ll take baseline test scores from participants. Then, your participants will undergo a 5-minute meditation exercise. Finally, you’ll record participants’ scores from a second math test.
In this experiment, the independent variable is the 5-minute meditation exercise, and the dependent variable is the math test score from before and after the intervention. Example: Correlational research design In a correlational study, you test whether there is a relationship between parental income and GPA in graduating college students. To collect your data, you will ask participants to fill in a survey and self-report their parents’ incomes and their own GPA.
Measuring variables
When planning a research design, you should operationalize your variables and decide exactly how you will measure them.
For statistical analysis, it’s important to consider the level of measurement of your variables, which tells you what kind of data they contain:
- Categorical data represents groupings. These may be nominal (e.g., gender) or ordinal (e.g. level of language ability).
- Quantitative data represents amounts. These may be on an interval scale (e.g. test score) or a ratio scale (e.g. age).
Many variables can be measured at different levels of precision. For example, age data can be quantitative (8 years old) or categorical (young). If a variable is coded numerically (e.g., level of agreement from 1–5), it doesn’t automatically mean that it’s quantitative instead of categorical.
Identifying the measurement level is important for choosing appropriate statistics and hypothesis tests. For example, you can calculate a mean score with quantitative data, but not with categorical data.
In a research study, along with measures of your variables of interest, you’ll often collect data on relevant participant characteristics.
Variable | Type of data |
---|---|
Age | Quantitative (ratio) |
Gender | Categorical (nominal) |
Race or ethnicity | Categorical (nominal) |
Baseline test scores | Quantitative (interval) |
Final test scores | Quantitative (interval) |
Parental income | Quantitative (ratio) |
---|---|
GPA | Quantitative (interval) |
Prevent plagiarism. Run a free check.
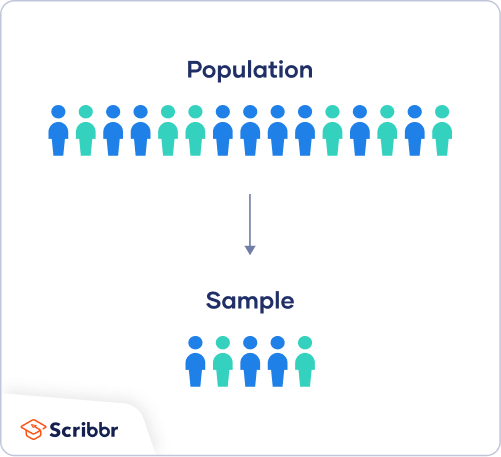
In most cases, it’s too difficult or expensive to collect data from every member of the population you’re interested in studying. Instead, you’ll collect data from a sample.
Statistical analysis allows you to apply your findings beyond your own sample as long as you use appropriate sampling procedures . You should aim for a sample that is representative of the population.
Sampling for statistical analysis
There are two main approaches to selecting a sample.
- Probability sampling: every member of the population has a chance of being selected for the study through random selection.
- Non-probability sampling: some members of the population are more likely than others to be selected for the study because of criteria such as convenience or voluntary self-selection.
In theory, for highly generalizable findings, you should use a probability sampling method. Random selection reduces several types of research bias , like sampling bias , and ensures that data from your sample is actually typical of the population. Parametric tests can be used to make strong statistical inferences when data are collected using probability sampling.
But in practice, it’s rarely possible to gather the ideal sample. While non-probability samples are more likely to at risk for biases like self-selection bias , they are much easier to recruit and collect data from. Non-parametric tests are more appropriate for non-probability samples, but they result in weaker inferences about the population.
If you want to use parametric tests for non-probability samples, you have to make the case that:
- your sample is representative of the population you’re generalizing your findings to.
- your sample lacks systematic bias.
Keep in mind that external validity means that you can only generalize your conclusions to others who share the characteristics of your sample. For instance, results from Western, Educated, Industrialized, Rich and Democratic samples (e.g., college students in the US) aren’t automatically applicable to all non-WEIRD populations.
If you apply parametric tests to data from non-probability samples, be sure to elaborate on the limitations of how far your results can be generalized in your discussion section .
Create an appropriate sampling procedure
Based on the resources available for your research, decide on how you’ll recruit participants.
- Will you have resources to advertise your study widely, including outside of your university setting?
- Will you have the means to recruit a diverse sample that represents a broad population?
- Do you have time to contact and follow up with members of hard-to-reach groups?
Your participants are self-selected by their schools. Although you’re using a non-probability sample, you aim for a diverse and representative sample. Example: Sampling (correlational study) Your main population of interest is male college students in the US. Using social media advertising, you recruit senior-year male college students from a smaller subpopulation: seven universities in the Boston area.
Calculate sufficient sample size
Before recruiting participants, decide on your sample size either by looking at other studies in your field or using statistics. A sample that’s too small may be unrepresentative of the sample, while a sample that’s too large will be more costly than necessary.
There are many sample size calculators online. Different formulas are used depending on whether you have subgroups or how rigorous your study should be (e.g., in clinical research). As a rule of thumb, a minimum of 30 units or more per subgroup is necessary.
To use these calculators, you have to understand and input these key components:
- Significance level (alpha): the risk of rejecting a true null hypothesis that you are willing to take, usually set at 5%.
- Statistical power : the probability of your study detecting an effect of a certain size if there is one, usually 80% or higher.
- Expected effect size : a standardized indication of how large the expected result of your study will be, usually based on other similar studies.
- Population standard deviation: an estimate of the population parameter based on a previous study or a pilot study of your own.
Once you’ve collected all of your data, you can inspect them and calculate descriptive statistics that summarize them.
Inspect your data
There are various ways to inspect your data, including the following:
- Organizing data from each variable in frequency distribution tables .
- Displaying data from a key variable in a bar chart to view the distribution of responses.
- Visualizing the relationship between two variables using a scatter plot .
By visualizing your data in tables and graphs, you can assess whether your data follow a skewed or normal distribution and whether there are any outliers or missing data.
A normal distribution means that your data are symmetrically distributed around a center where most values lie, with the values tapering off at the tail ends.
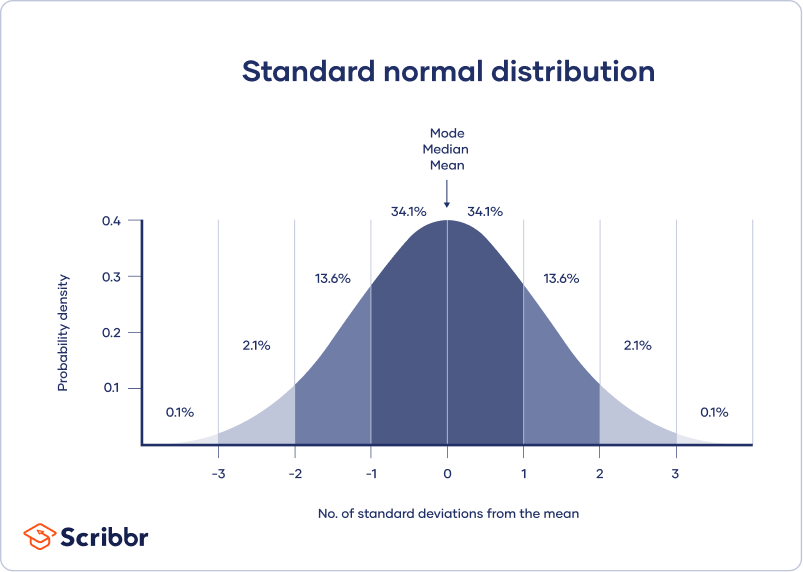
In contrast, a skewed distribution is asymmetric and has more values on one end than the other. The shape of the distribution is important to keep in mind because only some descriptive statistics should be used with skewed distributions.
Extreme outliers can also produce misleading statistics, so you may need a systematic approach to dealing with these values.
Calculate measures of central tendency
Measures of central tendency describe where most of the values in a data set lie. Three main measures of central tendency are often reported:
- Mode : the most popular response or value in the data set.
- Median : the value in the exact middle of the data set when ordered from low to high.
- Mean : the sum of all values divided by the number of values.
However, depending on the shape of the distribution and level of measurement, only one or two of these measures may be appropriate. For example, many demographic characteristics can only be described using the mode or proportions, while a variable like reaction time may not have a mode at all.
Calculate measures of variability
Measures of variability tell you how spread out the values in a data set are. Four main measures of variability are often reported:
- Range : the highest value minus the lowest value of the data set.
- Interquartile range : the range of the middle half of the data set.
- Standard deviation : the average distance between each value in your data set and the mean.
- Variance : the square of the standard deviation.
Once again, the shape of the distribution and level of measurement should guide your choice of variability statistics. The interquartile range is the best measure for skewed distributions, while standard deviation and variance provide the best information for normal distributions.
Using your table, you should check whether the units of the descriptive statistics are comparable for pretest and posttest scores. For example, are the variance levels similar across the groups? Are there any extreme values? If there are, you may need to identify and remove extreme outliers in your data set or transform your data before performing a statistical test.
Pretest scores | Posttest scores | |
---|---|---|
Mean | 68.44 | 75.25 |
Standard deviation | 9.43 | 9.88 |
Variance | 88.96 | 97.96 |
Range | 36.25 | 45.12 |
30 |
From this table, we can see that the mean score increased after the meditation exercise, and the variances of the two scores are comparable. Next, we can perform a statistical test to find out if this improvement in test scores is statistically significant in the population. Example: Descriptive statistics (correlational study) After collecting data from 653 students, you tabulate descriptive statistics for annual parental income and GPA.
It’s important to check whether you have a broad range of data points. If you don’t, your data may be skewed towards some groups more than others (e.g., high academic achievers), and only limited inferences can be made about a relationship.
Parental income (USD) | GPA | |
---|---|---|
Mean | 62,100 | 3.12 |
Standard deviation | 15,000 | 0.45 |
Variance | 225,000,000 | 0.16 |
Range | 8,000–378,000 | 2.64–4.00 |
653 |
A number that describes a sample is called a statistic , while a number describing a population is called a parameter . Using inferential statistics , you can make conclusions about population parameters based on sample statistics.
Researchers often use two main methods (simultaneously) to make inferences in statistics.
- Estimation: calculating population parameters based on sample statistics.
- Hypothesis testing: a formal process for testing research predictions about the population using samples.
You can make two types of estimates of population parameters from sample statistics:
- A point estimate : a value that represents your best guess of the exact parameter.
- An interval estimate : a range of values that represent your best guess of where the parameter lies.
If your aim is to infer and report population characteristics from sample data, it’s best to use both point and interval estimates in your paper.
You can consider a sample statistic a point estimate for the population parameter when you have a representative sample (e.g., in a wide public opinion poll, the proportion of a sample that supports the current government is taken as the population proportion of government supporters).
There’s always error involved in estimation, so you should also provide a confidence interval as an interval estimate to show the variability around a point estimate.
A confidence interval uses the standard error and the z score from the standard normal distribution to convey where you’d generally expect to find the population parameter most of the time.
Hypothesis testing
Using data from a sample, you can test hypotheses about relationships between variables in the population. Hypothesis testing starts with the assumption that the null hypothesis is true in the population, and you use statistical tests to assess whether the null hypothesis can be rejected or not.
Statistical tests determine where your sample data would lie on an expected distribution of sample data if the null hypothesis were true. These tests give two main outputs:
- A test statistic tells you how much your data differs from the null hypothesis of the test.
- A p value tells you the likelihood of obtaining your results if the null hypothesis is actually true in the population.
Statistical tests come in three main varieties:
- Comparison tests assess group differences in outcomes.
- Regression tests assess cause-and-effect relationships between variables.
- Correlation tests assess relationships between variables without assuming causation.
Your choice of statistical test depends on your research questions, research design, sampling method, and data characteristics.
Parametric tests
Parametric tests make powerful inferences about the population based on sample data. But to use them, some assumptions must be met, and only some types of variables can be used. If your data violate these assumptions, you can perform appropriate data transformations or use alternative non-parametric tests instead.
A regression models the extent to which changes in a predictor variable results in changes in outcome variable(s).
- A simple linear regression includes one predictor variable and one outcome variable.
- A multiple linear regression includes two or more predictor variables and one outcome variable.
Comparison tests usually compare the means of groups. These may be the means of different groups within a sample (e.g., a treatment and control group), the means of one sample group taken at different times (e.g., pretest and posttest scores), or a sample mean and a population mean.
- A t test is for exactly 1 or 2 groups when the sample is small (30 or less).
- A z test is for exactly 1 or 2 groups when the sample is large.
- An ANOVA is for 3 or more groups.
The z and t tests have subtypes based on the number and types of samples and the hypotheses:
- If you have only one sample that you want to compare to a population mean, use a one-sample test .
- If you have paired measurements (within-subjects design), use a dependent (paired) samples test .
- If you have completely separate measurements from two unmatched groups (between-subjects design), use an independent (unpaired) samples test .
- If you expect a difference between groups in a specific direction, use a one-tailed test .
- If you don’t have any expectations for the direction of a difference between groups, use a two-tailed test .
The only parametric correlation test is Pearson’s r . The correlation coefficient ( r ) tells you the strength of a linear relationship between two quantitative variables.
However, to test whether the correlation in the sample is strong enough to be important in the population, you also need to perform a significance test of the correlation coefficient, usually a t test, to obtain a p value. This test uses your sample size to calculate how much the correlation coefficient differs from zero in the population.
You use a dependent-samples, one-tailed t test to assess whether the meditation exercise significantly improved math test scores. The test gives you:
- a t value (test statistic) of 3.00
- a p value of 0.0028
Although Pearson’s r is a test statistic, it doesn’t tell you anything about how significant the correlation is in the population. You also need to test whether this sample correlation coefficient is large enough to demonstrate a correlation in the population.
A t test can also determine how significantly a correlation coefficient differs from zero based on sample size. Since you expect a positive correlation between parental income and GPA, you use a one-sample, one-tailed t test. The t test gives you:
- a t value of 3.08
- a p value of 0.001
Here's why students love Scribbr's proofreading services
Discover proofreading & editing
The final step of statistical analysis is interpreting your results.
Statistical significance
In hypothesis testing, statistical significance is the main criterion for forming conclusions. You compare your p value to a set significance level (usually 0.05) to decide whether your results are statistically significant or non-significant.
Statistically significant results are considered unlikely to have arisen solely due to chance. There is only a very low chance of such a result occurring if the null hypothesis is true in the population.
This means that you believe the meditation intervention, rather than random factors, directly caused the increase in test scores. Example: Interpret your results (correlational study) You compare your p value of 0.001 to your significance threshold of 0.05. With a p value under this threshold, you can reject the null hypothesis. This indicates a statistically significant correlation between parental income and GPA in male college students.
Note that correlation doesn’t always mean causation, because there are often many underlying factors contributing to a complex variable like GPA. Even if one variable is related to another, this may be because of a third variable influencing both of them, or indirect links between the two variables.
Effect size
A statistically significant result doesn’t necessarily mean that there are important real life applications or clinical outcomes for a finding.
In contrast, the effect size indicates the practical significance of your results. It’s important to report effect sizes along with your inferential statistics for a complete picture of your results. You should also report interval estimates of effect sizes if you’re writing an APA style paper .
With a Cohen’s d of 0.72, there’s medium to high practical significance to your finding that the meditation exercise improved test scores. Example: Effect size (correlational study) To determine the effect size of the correlation coefficient, you compare your Pearson’s r value to Cohen’s effect size criteria.
Decision errors
Type I and Type II errors are mistakes made in research conclusions. A Type I error means rejecting the null hypothesis when it’s actually true, while a Type II error means failing to reject the null hypothesis when it’s false.
You can aim to minimize the risk of these errors by selecting an optimal significance level and ensuring high power . However, there’s a trade-off between the two errors, so a fine balance is necessary.
Frequentist versus Bayesian statistics
Traditionally, frequentist statistics emphasizes null hypothesis significance testing and always starts with the assumption of a true null hypothesis.
However, Bayesian statistics has grown in popularity as an alternative approach in the last few decades. In this approach, you use previous research to continually update your hypotheses based on your expectations and observations.
Bayes factor compares the relative strength of evidence for the null versus the alternative hypothesis rather than making a conclusion about rejecting the null hypothesis or not.
If you want to know more about statistics , methodology , or research bias , make sure to check out some of our other articles with explanations and examples.
- Student’s t -distribution
- Normal distribution
- Null and Alternative Hypotheses
- Chi square tests
- Confidence interval
Methodology
- Cluster sampling
- Stratified sampling
- Data cleansing
- Reproducibility vs Replicability
- Peer review
- Likert scale
Research bias
- Implicit bias
- Framing effect
- Cognitive bias
- Placebo effect
- Hawthorne effect
- Hostile attribution bias
- Affect heuristic
Is this article helpful?
Other students also liked.
- Descriptive Statistics | Definitions, Types, Examples
- Inferential Statistics | An Easy Introduction & Examples
- Choosing the Right Statistical Test | Types & Examples
More interesting articles
- Akaike Information Criterion | When & How to Use It (Example)
- An Easy Introduction to Statistical Significance (With Examples)
- An Introduction to t Tests | Definitions, Formula and Examples
- ANOVA in R | A Complete Step-by-Step Guide with Examples
- Central Limit Theorem | Formula, Definition & Examples
- Central Tendency | Understanding the Mean, Median & Mode
- Chi-Square (Χ²) Distributions | Definition & Examples
- Chi-Square (Χ²) Table | Examples & Downloadable Table
- Chi-Square (Χ²) Tests | Types, Formula & Examples
- Chi-Square Goodness of Fit Test | Formula, Guide & Examples
- Chi-Square Test of Independence | Formula, Guide & Examples
- Coefficient of Determination (R²) | Calculation & Interpretation
- Correlation Coefficient | Types, Formulas & Examples
- Frequency Distribution | Tables, Types & Examples
- How to Calculate Standard Deviation (Guide) | Calculator & Examples
- How to Calculate Variance | Calculator, Analysis & Examples
- How to Find Degrees of Freedom | Definition & Formula
- How to Find Interquartile Range (IQR) | Calculator & Examples
- How to Find Outliers | 4 Ways with Examples & Explanation
- How to Find the Geometric Mean | Calculator & Formula
- How to Find the Mean | Definition, Examples & Calculator
- How to Find the Median | Definition, Examples & Calculator
- How to Find the Mode | Definition, Examples & Calculator
- How to Find the Range of a Data Set | Calculator & Formula
- Hypothesis Testing | A Step-by-Step Guide with Easy Examples
- Interval Data and How to Analyze It | Definitions & Examples
- Levels of Measurement | Nominal, Ordinal, Interval and Ratio
- Linear Regression in R | A Step-by-Step Guide & Examples
- Missing Data | Types, Explanation, & Imputation
- Multiple Linear Regression | A Quick Guide (Examples)
- Nominal Data | Definition, Examples, Data Collection & Analysis
- Normal Distribution | Examples, Formulas, & Uses
- Null and Alternative Hypotheses | Definitions & Examples
- One-way ANOVA | When and How to Use It (With Examples)
- Ordinal Data | Definition, Examples, Data Collection & Analysis
- Parameter vs Statistic | Definitions, Differences & Examples
- Pearson Correlation Coefficient (r) | Guide & Examples
- Poisson Distributions | Definition, Formula & Examples
- Probability Distribution | Formula, Types, & Examples
- Quartiles & Quantiles | Calculation, Definition & Interpretation
- Ratio Scales | Definition, Examples, & Data Analysis
- Simple Linear Regression | An Easy Introduction & Examples
- Skewness | Definition, Examples & Formula
- Statistical Power and Why It Matters | A Simple Introduction
- Student's t Table (Free Download) | Guide & Examples
- T-distribution: What it is and how to use it
- Test statistics | Definition, Interpretation, and Examples
- The Standard Normal Distribution | Calculator, Examples & Uses
- Two-Way ANOVA | Examples & When To Use It
- Type I & Type II Errors | Differences, Examples, Visualizations
- Understanding Confidence Intervals | Easy Examples & Formulas
- Understanding P values | Definition and Examples
- Variability | Calculating Range, IQR, Variance, Standard Deviation
- What is Effect Size and Why Does It Matter? (Examples)
- What Is Kurtosis? | Definition, Examples & Formula
- What Is Standard Error? | How to Calculate (Guide with Examples)
What is your plagiarism score?
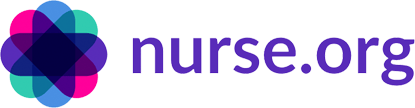
What’s Really Behind the Nursing Shortage? 1,500 Nurses Share Their Stories
- Survey Results
- What Is the Nursing Shortage?
- Reasons Nurses Quit
- Hope For the Future
- What Nurses Need Now
- How to Take Action
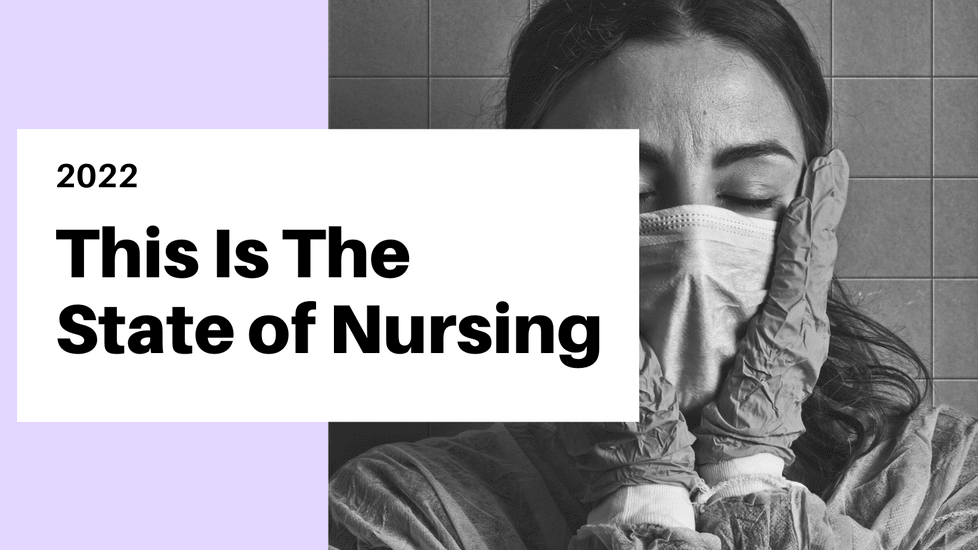
Winner of the Gold Award for the Digital Health Awards, Best Media/Publications Article, Spring 2022
Update 10/10/2022
The findings of Nurse.org's 2021 State of Nursing Survey revealed some harsh truths about the profession but also spoke to the strength, perseverance, and passion that nurses have for their work. Nurse.org has relaunched the State of Nursing survey in 2022 with the aim to capture a complete picture of the true state of the profession - from how nurses feel about work, how nurses are being treated, how nurses feel about the future of nursing, nurse's mental wellbeing and what nurses think needs to change within the profession. Take the survey now (it takes less than 10 minutes.)
>> Take Nurse.org's NEW 2022 "State of Nursing Survey" and let your voice be heard about issues in nursing that matter most.
January 26, 2022
If the past two years have taught the world anything, it's that nurses are NOT okay. The truth is that despite the 7 pm cheers, the commercials thanking nurses for their dedication and selflessness, and the free food from major retailers – the overwhelming majority of nurses are burnt out, underpaid, overworked, and underappreciated.
With millions of nurses worldwide, Nurse.org wanted to truly understand the current state of nursing and give nurses a voice to share their thoughts, feelings, and apprehensions about the nursing profession. We surveyed nearly 1,500 nurses to find out how they felt about the past year and get to the real reasons behind the nursing shortage . The responses were heartbreaking, but not without hope.
What We Found: Nurses Are Struggling
Nurses are struggling. Regardless of practice specialty, age, or state of practice – the answers were all the same. Nurses, NPs, and APRNs are all struggling and need help.
Only 12% of the nurses surveyed are happy where they are and interestingly, 36% would like to stay in their current positions but changes would need to be made for that to happen. Nurses report wanting safe staffing, safer patient ratio assignments, and increased pay in order to stay in their current roles.
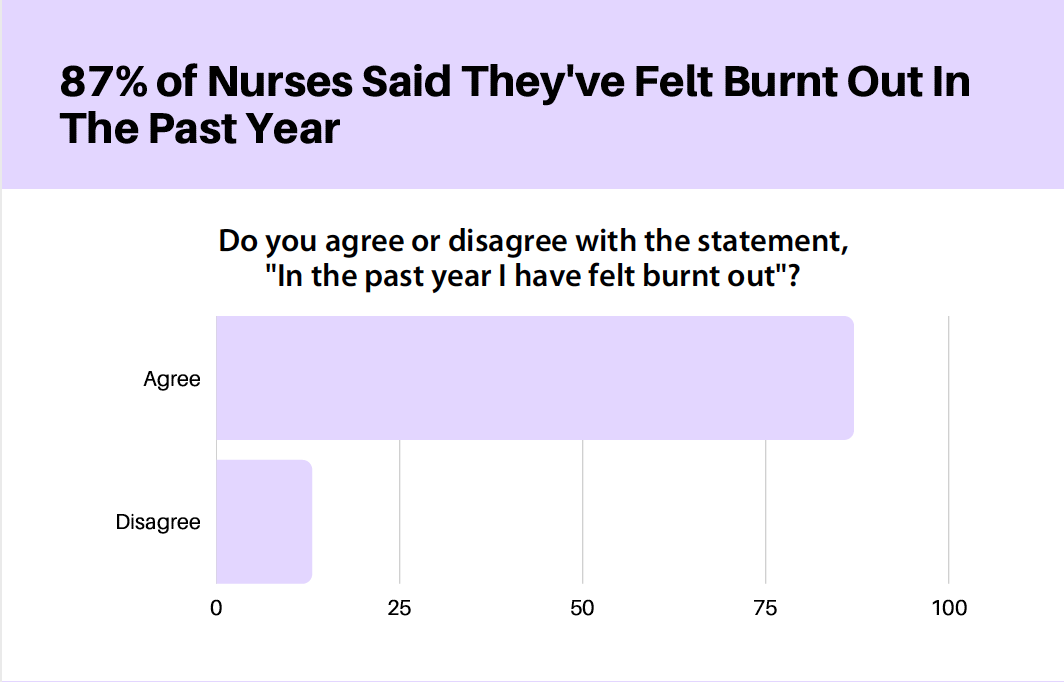
Nurses didn’t hold back when discussing their feelings regarding the current state of nursing:
- 87% feel burnt out
- 84% are frustrated with administrators
- 84% feel they are underpaid
- 83% feel their mental health has suffered
- 77% feel unsupported at work
- 61% feel unappreciated
- 60% have felt uncomfortable having to work outside of their comfort zone in the past year
- 58% of nurses have felt frustrated with their patients
- 58% of nurses have felt unsafe at work in the past year
The numbers don’t lie. It’s astounding that a profession continually recognized for its compassion, strength, and resilience is suffering . And the suffering is universal.
One nurse responded with the following, “I have been an RN for 34 years and in my specialty of nursing for 31 years and I am burned out.”
What Is the Nursing Shortage and Why is it Happening?
You’ve likely heard about the nursing shortage, but what does that mean and why is it happening?
According to the U.S. Bureau of Labor Statistics (BLS) , the employment of registered nurses is projected to grow 9% from 2020 to 2030. Approximately 194,500 openings for registered nurses are projected each year, on average, over the decade. However, this number was projected prior to the pandemic, and before the mass exodus of bedside clinical nurses. As a result, it’s likely substantially lower than what the real demand for nurses will look like.
The American Nurses Association (ANA) reports that the increased need for nurses spans beyond the current pandemic. In fact, they sent a letter to the U.S. Department of Health and Human Services (HHS) on September 1, 2021, urging the country to declare the current and unsustainable nurse staffing shortage to be a national crisis.
The ANA attributes the needs for thousands of nurses to the following:
- The Affordable Care Act made access to health care services possible for more people
- Increased focus “primary care, prevention, wellness, and chronic disease management”
- Aging baby-boomer population
- Growing interest in community-based care
Why Are Nurses Really Leaving The Bedside?
However, those stats don’t address some of the systemic issues nurses face every day, particularly in the midst of a pandemic. That’s why we asked nurses why they are really leaving the bedside.
What we heard is that, overwhelmingly, the number one reason nurses want to leave the bedside is because of unsafe staffing ratios. This leads to a never-ending cycle of shortages: nurses face unsafe staffing ratios so they decide to leave the bedside, this results in even fewer nurses available to care for patients, so the downward cycle continues.
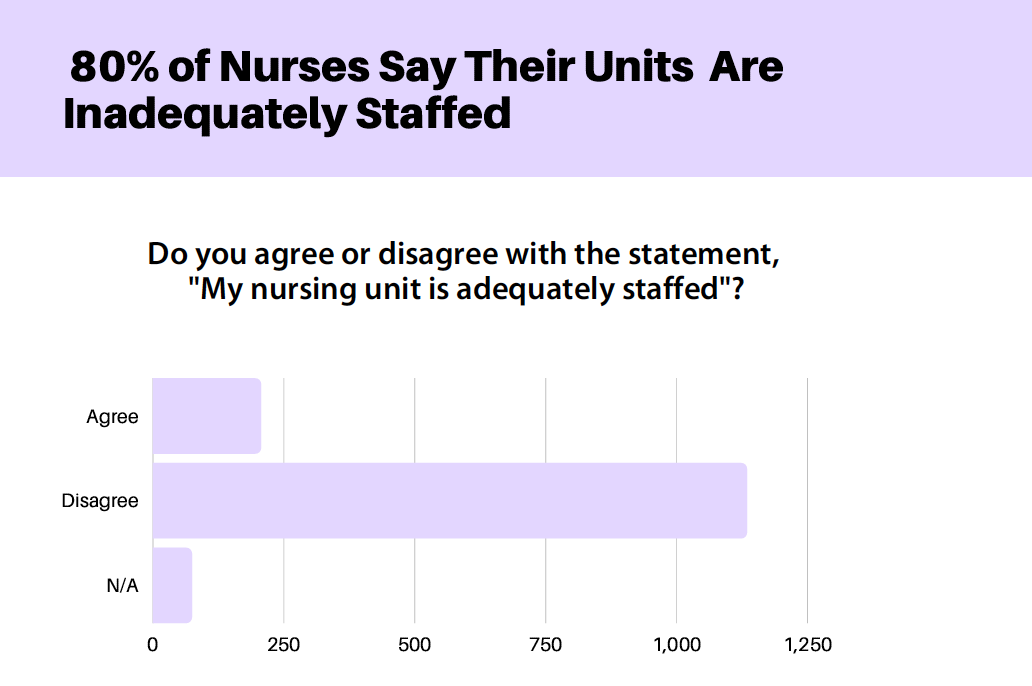
Essentially, nurses are dealing with an increased workload with fewer resources. Typically, pre-covid ICU nurses would experience a 1:1 or 2:1 patient-to-nurse ratio. Now ICU nurses throughout the country are experiencing a 3:1 or 4:1 patient-to-nurse ratio which exacerbates staff burnout and unsafe nursing practices.
One nurse reported, “With increased patient census, staffing ratios are very unsafe especially with high acuity patients. Having 4+ critically ill patients not only puts licenses at risk but the patients do not benefit at all. We’re just running around doing tasks, not providing adequate care.”
Unsafe Staffing Ratios Are Just Part of the Problem
While a big piece of the puzzle, unsafe staffing issues are, unfortunately, one part of a long list of issues plaguing nurses today.
Nurses are leaving the bedside because of issues like:
- Inadequate staffing ratios
- Not getting equal pay for equal experience
- Not receiving hazard pay during a pandemic
- Not having adequate back up
- An inability to take breaks, sick days, or even turn down extra shifts
To learn more about the nursing shortage and learn ways you can get involved, check out the full report here .
Despite All This, Nurses Still Have Hope
70% of nurses still think that nursing is a great career and 64% still think that new nurses should join the profession.
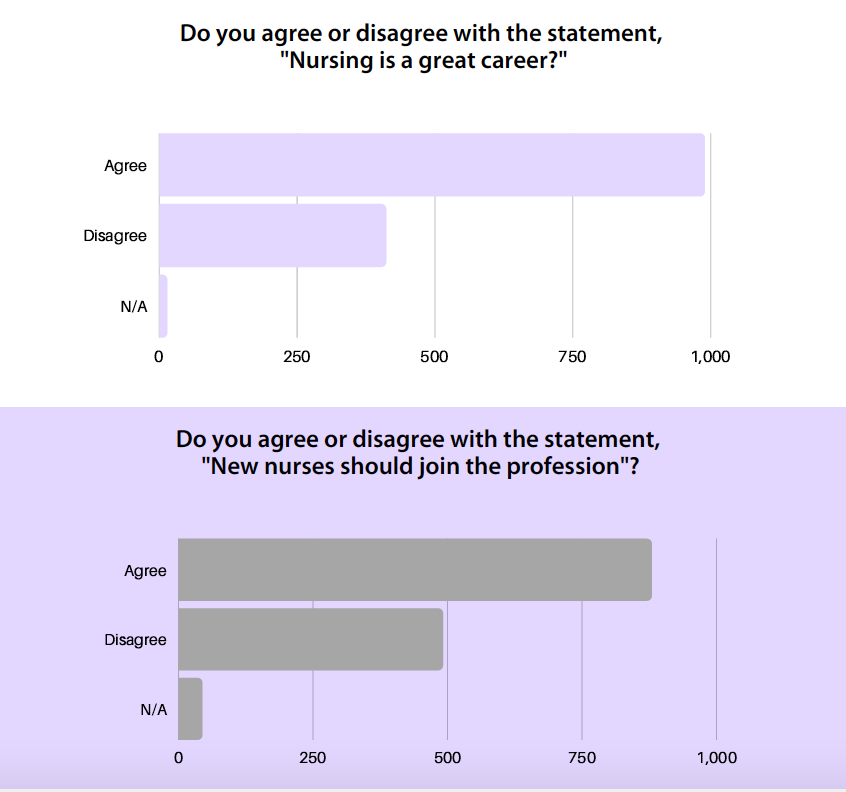
“If you’re a student considering becoming a nurse, please know that you are not walking into a doomed profession. You will never meet anyone who is more determined, more resourceful, or more ready to jump in and lend a helping hand than a nurse."
--– Nurse Alice Benjamin, MSN, APRN, ACNS-BC, FNP-C, CCRN, CEN, CV-BC, Chief Nursing Officer and Correspondent at Nurse.org
If you’re a nurse, you know that nursing isn’t just a profession, it’s a calling. It’s devastating to see that so many nurses are suffering in their quest to heal and give care, but it’s heartening to know they are not without hope.
What Nurses Need Now
If you’re a nurse, know that your job is simply to put yourself first. If we want to solve the nursing shortage (and we do!), it can't happen without nurses recognizing that they are NOT the problem.
"The problem is not with nurses or nursing; the problem is that nurses have been so busy taking care of others that no one has taken care of them. And we’re here to change that--and by entering the nursing profession, you will be part of the solution too”
– Nurse Alice Benjamin, MSN, APRN, ACNS-BC, FNP-C, CCRN, CEN, CV-BC, Chief Nursing Officer and Correspondent at Nurse.org
The truth is nurses need a lot more to be incentivized to stay practicing clinically at the bedside. Nurses reported needing:
- Higher pay
- Safe nurse-to-patient ratios
- Hazard pay
- REAL mental health resources
- Adequate staff support
- Support programs for new nurses
4 Ways to Support Nurses and Take Action
While we may not be able to make this change at an individual level, collectively, we can amplify the voice of nurses and shed some light on the issues that they are facing every day. Together, we have the power to create meaningful, lasting change for current and future nurses. Here's how to get involved:
1. Sign the Pledge
Sign the pledge seen below and encourage your friends & colleagues to do the same. While you’re at it, print it out and post it in your break room.
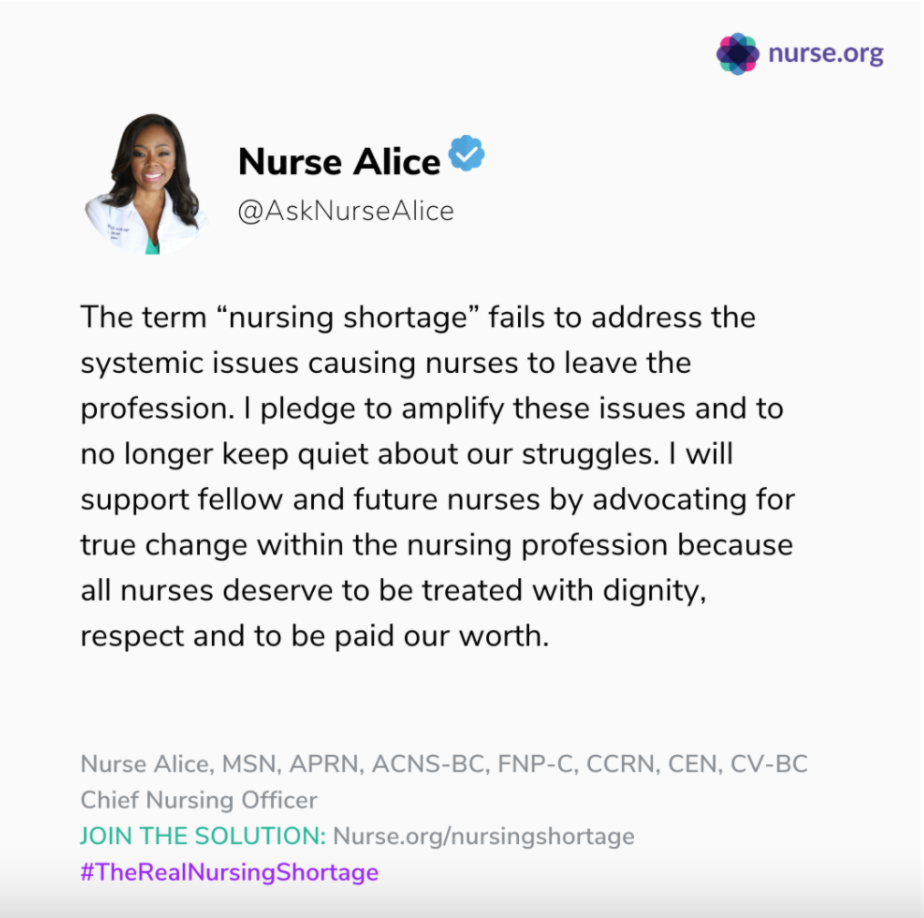
2. Spread the Word
Change can’t happen unless we get the word out about what’s really going on. Share what you’ve heard and what you’ve experienced, and encourage others to do the same.
3. Contact Your Elected Officials
It’s time for elected officials to stand up for nurses. Write them a letter. Call their office. Demand change for nurses. Click here to get the contact information for your local and state Officials.
4. Download and Share the Report
Get even more in-depth insights into what’s going on with the state of nursing and the issues that nurses face today, click here to download the full State of Nursing report or read about the best and worst specialties for nurses during COVID .
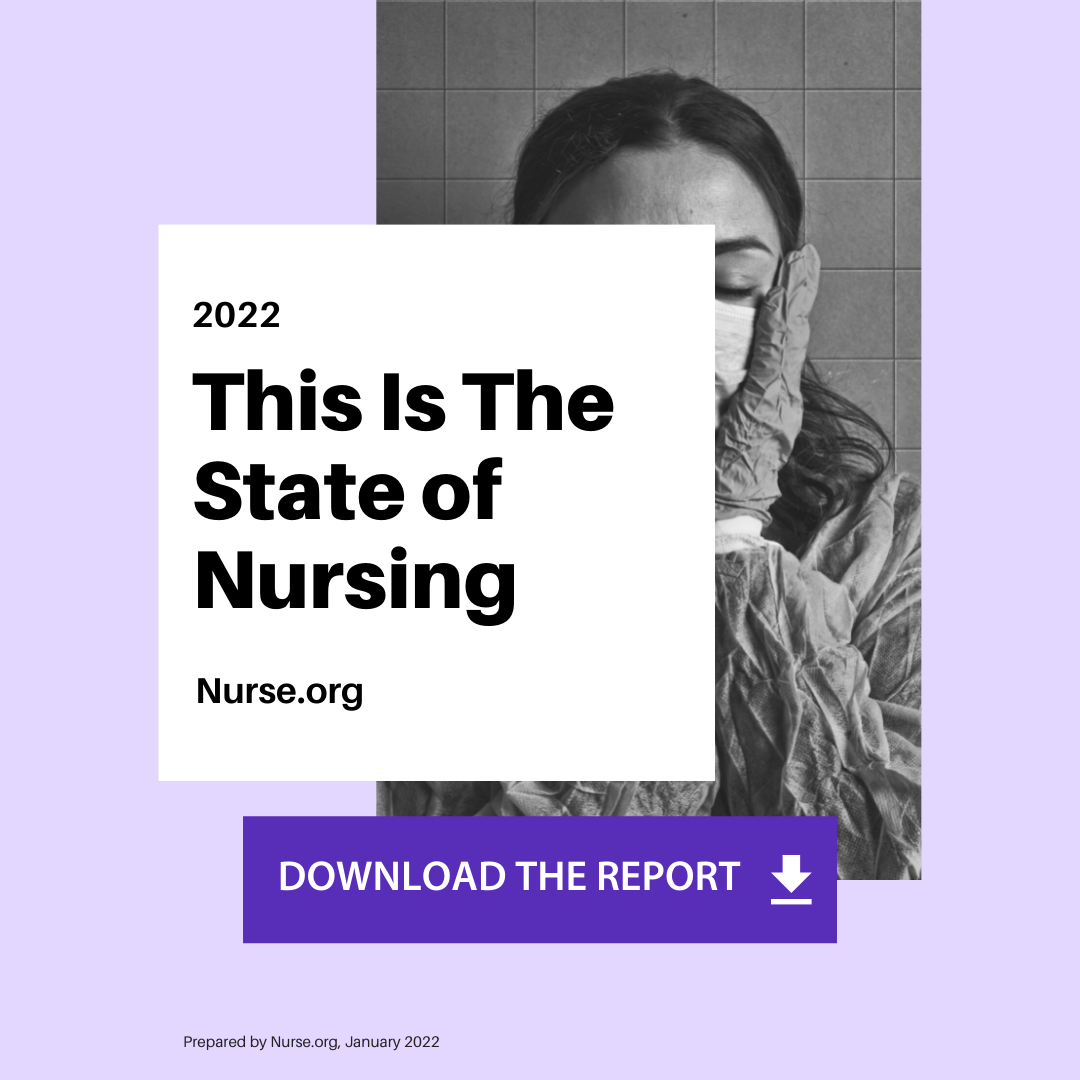
“If you are a current nurse considering leaving the profession, be assured that you are not alone in your struggles. If all you’ve had the energy for is keeping your head down and getting through your shifts, sleeping, and getting up to do it all over again, know that you are doing enough. It’s not your responsibility to solve the nursing shortage.”
– Nurse Alice Benjamin, MSN, APRN, ACNS-BC, FNP-C, CCRN, CEN, CV-BC, Chief Nursing Officer and Correspondent at Nurse.org
Find Nursing Programs
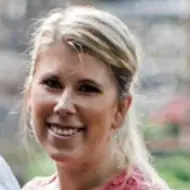
Kathleen Gaines (nee Colduvell) is a nationally published writer turned Pediatric ICU nurse from Philadelphia with over 13 years of ICU experience. She has an extensive ICU background having formerly worked in the CICU and NICU at several major hospitals in the Philadelphia region. After earning her MSN in Education from Loyola University of New Orleans, she currently also teaches for several prominent Universities making sure the next generation is ready for the bedside. As a certified breastfeeding counselor and trauma certified nurse, she is always ready for the next nursing challenge.
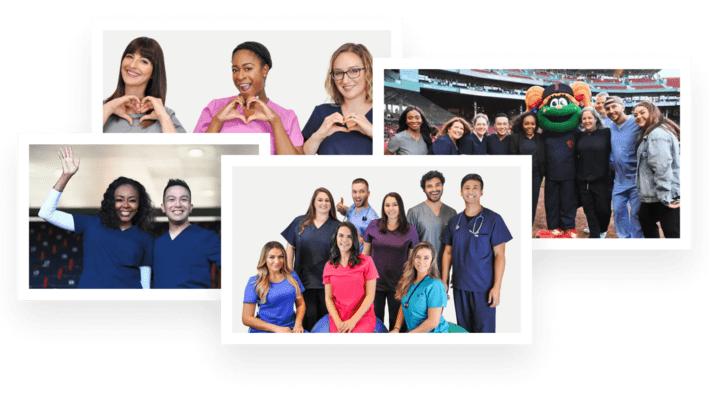
Plus, get exclusive access to discounts for nurses, stay informed on the latest nurse news, and learn how to take the next steps in your career.
By clicking “Join Now”, you agree to receive email newsletters and special offers from Nurse.org. You may unsubscribe at any time by using the unsubscribe link, found at the bottom of every email.

IMAGES
VIDEO
COMMENTS
The field of statistics is concerned with collecting, analyzing, interpreting, and presenting data.. In the field of nursing, statistics is important for the following reasons: Reason 1: Statistics allows nurses to understand how to interpret descriptive statistics like mean, median, standard deviation, range, and percentiles.. Reason 2: Statistics allows nurses to understand how to interpret ...
As a nurse, you will use statistics to identify specific patterns in important signs and symptoms and be able to respond better to any medical changes to your patient. Using frequency charts or data sheets to document the timing of medication given to patients is another way that nurses use statistics. Support evidence-based nursing practices ...
The research analysis demonstrates the use of three types of descriptive statistics. First, survey results for 154 participants were presented in terms of frequencies to reflect the number of times variables occurred (Table 1) (Tudor, Berger, Polivka, Chlebowy, & Thomas, 2014). Second, the use of the mean also shows descriptive statistics.
REASON #3: Statistics Help Identify Public Health Risks. Another top reason statistics is important in nursing is that public health nurses can use health statistics to understand risk factors affecting various populations or communities. By understanding risk factors, they can then track and monitor disease events, such as how the COVID-19 ...
It's calculated by taking the square root of the sum of all numbers minus the mean (squared) and dividing by one less than the number of values. For example, in a data set of five systolic blood pressures of 125, 128, 142, 145, and 150, the mean would be 138, based on this calculation: (125+128+142+145+150)/5.
Common descriptive statistics. The most common types of descriptive statistics are the measures of central tendency (mean, median, and mode) that are used in most levels of math, research, evidence-based practice, and quality improvement. These measures describe the central portion of frequency distribution for a data set.
Descriptive Statistics Essay Example. I'm working on a Statistics question and need guidance to help me study. There is often the requirement to evaluate descriptive statistics for data within the organization or for health care information. Every year the National Cancer Institute collects and publishes data based on patient demographics.
A working understanding of the major fundamentals of statistical analysis is required to incorporate the findings of empirical research into nursing practice. The primary focus of this article is to describe common statistical terms, present some common statistical tests, and explain the interpretation of results from inferential statistics in nursing research. An overview of major concepts in ...
The application of statistical data to health care provides quality health care services by ensuring that none of the valid information is missing. In the same way, the application of statistical data offers safety for the patients. The research shows that statistical data could be used to measure drug safety alert response and defect adverse ...
Significance to Leadership. The employment of statistics within hospital settings and staff is crucial for the team's productivity and long-term planning. When it comes to statistical analysis, some of the most important cases of statistical data include patient ratio and retention, patient-to-bed ratio, and readmission rates.
The following are the general steps for statistical analysis: (1) formulate a hypothesis, (2) select an appropriate statistical test, (3) conduct a power analysis, (4) prepare data for analysis, (5) start with descriptive statistics, (6) check assumptions of tests, (7) run the analysis, (8) examine the statistical model, (9) report the results ...
Welcome to this focused issue on statistics in nursing research! Nursing Research put out a special call for papers on this topic in 2011. The response was significant and included 22 submitted manuscripts. Each submission went through a rigorous peer review process that included multiple statistical reviewers. This special issue presents 10 ...
The paper discusses statistics for nursing research. It includes mean age, percentage of patients who never used tobacco, the standard deviation for age, etc. IvyPanda® Free Essays
CHAPTER 4 STATISTICS INTRDUCTION Statistics is a branch of mathematics dealing with the collection, analysis, interpretation, presentation, and organization... Essays Topics
Essential tips and strategies for writing effective nursing school essays. The perfect guide for students seeking admission to nursing programs. Skip to content +1 (917) 781-1429 [email protected ... or facts and statistics if relevant. Conclude each paragraph with a sentence that ties the paragraph's main idea back to your overall thesis. ...
Increasing Quantitative Literacy in Nursing: A Joint Nursing-Statistician Perspective. Strong quantitative literacy is necessary to fulfill nurses' professional responsibilities across education levels, roles, and settings. Evidence-based practice and systems improvement are not possible if nurses do not understand the statistics employed in ...
Follow these 10 nursing school tips for a more impactful essay. 1. Read the Essay Guidelines Thoroughly. Following the directions is essential, as every nursing school establishes its own nursing school essay policies, topics and procedures. For example, you may need to write within a minimum or maximum word count range, and you'll likely ...
The application of statistics in health care is imperative to the attainment of a safe and effective healthcare environment. Healthcare facilities and organizations are particularly reliant on assessments and data to identify safety concerns and effectively address them. Statistics guarantees that decision-makers have the right data, in the ...
A nursing essay is a type of academic writing that aims to explore a particular topic related to nursing. It also presents a clear and concise argument or viewpoint supported by evidence. ... Provide some background information on the topic to help the reader better understand the context of your essay. This can include relevant statistics ...
In a 750-1,000 word paper, discuss the significance of statistical application in health care. Include the following: Describe the application of statistics in health care. Specifically discuss its significance to quality, safety, health promotion, and leadership. ( application of statistics in health care essay) Consider your organization or ...
Essay, Pages 12 (2883 words) Views. 7060. Statistics is a fundamental part of human knowledge. It is known to be the exact science of collection, interpretation, analysis and presentation of data. It is a mathematical science that gathers and explains causal phenomenon or relationship, analyzes and presents measurements, collects and analyzes ...
The Beginner's Guide to Statistical Analysis | 5 Steps & ...
84% feel they are underpaid. 83% feel their mental health has suffered. 77% feel unsupported at work. 61% feel unappreciated. 60% have felt uncomfortable having to work outside of their comfort zone in the past year. 58% of nurses have felt frustrated with their patients. 58% of nurses have felt unsafe at work in the past year.